From BI to enabling AI: How to transform your data team in 2020.
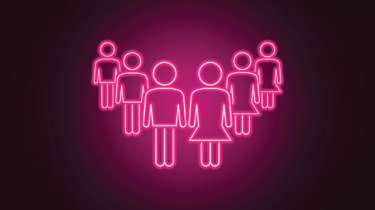
How can BI Managers, Heads of Data and other data leaders evolve a traditional BI team to meet the changing demands of the business? Ryan Moore, Head of Data and Analytics at Aiimi, explores.
We kicked off 2020 at Aiimi with a leadership workshop. Before the workshop, we collected data about the team and went through an exercise to determine our personality types. The objective was to help us understand each other and communicate better as a team. I came away from this with some new ideas about how to be more effective in my communication, but I also had to deal with the fact that I had an Eagle personality type. Eagles are results-oriented, impatient and don’t always explain what they want very well. Safe to say I tried to hide the results from my other half, as it confirmed what she’s been telling me for years.
So, what has all of this got to do with data?
- Organisations are constantly finding new ways to use data to help us do our jobs better.
- Those Eagle personality types sound a lot like the end customers of data teams - “I want the data and I want it now, but I can’t explain what I’m asking for.”
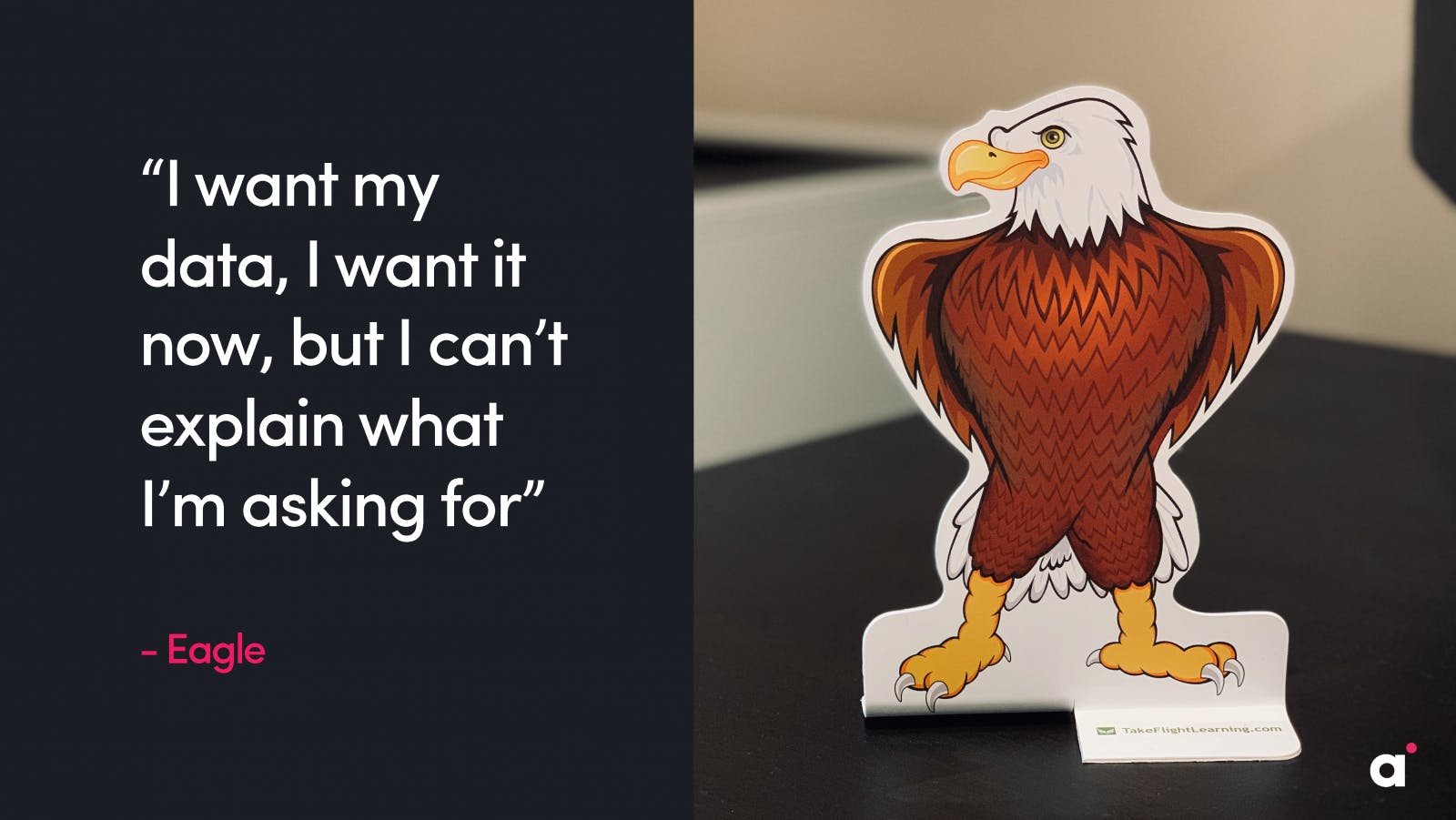
The data and analytics landscape is everchanging and the lovely folks at Gartner do an amazing job of keeping us on our toes with their magic quadrants and analytical trends. Still, one topic that I often find myself discussing with my peers in the industry is that the demands on data teams have fundamentally changed over the last few years. More specifically, demands are changing its role from a business intelligence (BI) function to a data provisioning and enabling function.
In larger organisations, this is down to the surge in self-service BI and having specialist teams for insight, data science and artificial intelligence. In small to medium size companies this is caused by departmental BI (using self-service BI tools or that spreadsheet software that everybody loves), built-in analytics, and AI functionality within existing business applications such as Salesforce. In both of these types of organisation, traditional BI teams are often faced with the decision to transform or risk getting sidelined.
I have been fortunate enough to take a customer’s data team on that journey over the past year, whilst also growing the data practice within Aiimi. And (like every good consultant!) I would love to share my lessons learnt with you, for free, sans the hype and confusing terminology.
Start with a vision
If you are lucky enough to work for an organisation that has a well-defined data strategy, then this is a great starting point to define your vision for the team. If a data strategy doesn’t exist, then the annual business plan is a good place to start. If you have neither of those, speak to the other heads of departments, gauge what they are struggling with and what they need to succeed –use that to influence your vision. The below is an example of how you could translate the objectives of a customer sales and marketing team into a vision for a data team:
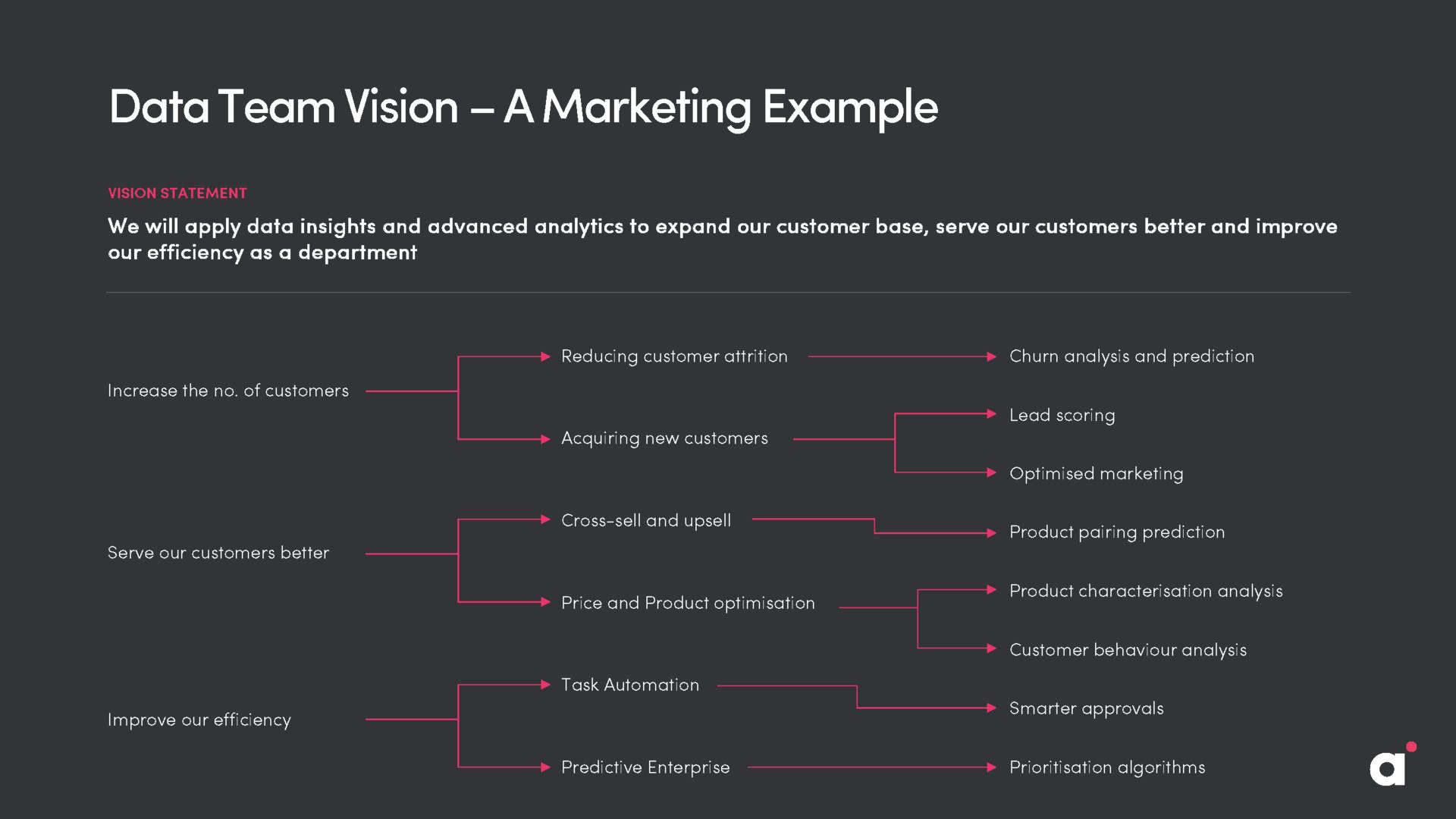
Blended vs. Specialist Teams
With your vision defined, the natural next step is to think about what resources you need to be able to deliver that vision. This process can vary by organisation depending on how far along they are in the data journey. If there are already departmental insight, analytics, data science or AI teams within the organisation then this choice is easy. Data teams can concentrate on provisioning good quality, trusted data while these specialist teams turn it into rich analytics, data science models or AI apps.
If you are part of an organisation running a centralised or combined BI and data science function, then you’ll want to look at the technical skills you need, depending on what you want to achieve as part of your vision. These may span roles like data architects, data engineers, platform engineers, data visualisation experts, data scientists and AI developers. While these roles sound very different from traditional BI roles (such as BI developer, ETL developer, BI administrator etc.) their function is similar, with the exception of data scientists and AI developers. The below image shows the key resource groups within a data team and the options of running blended vs specialist data teams.
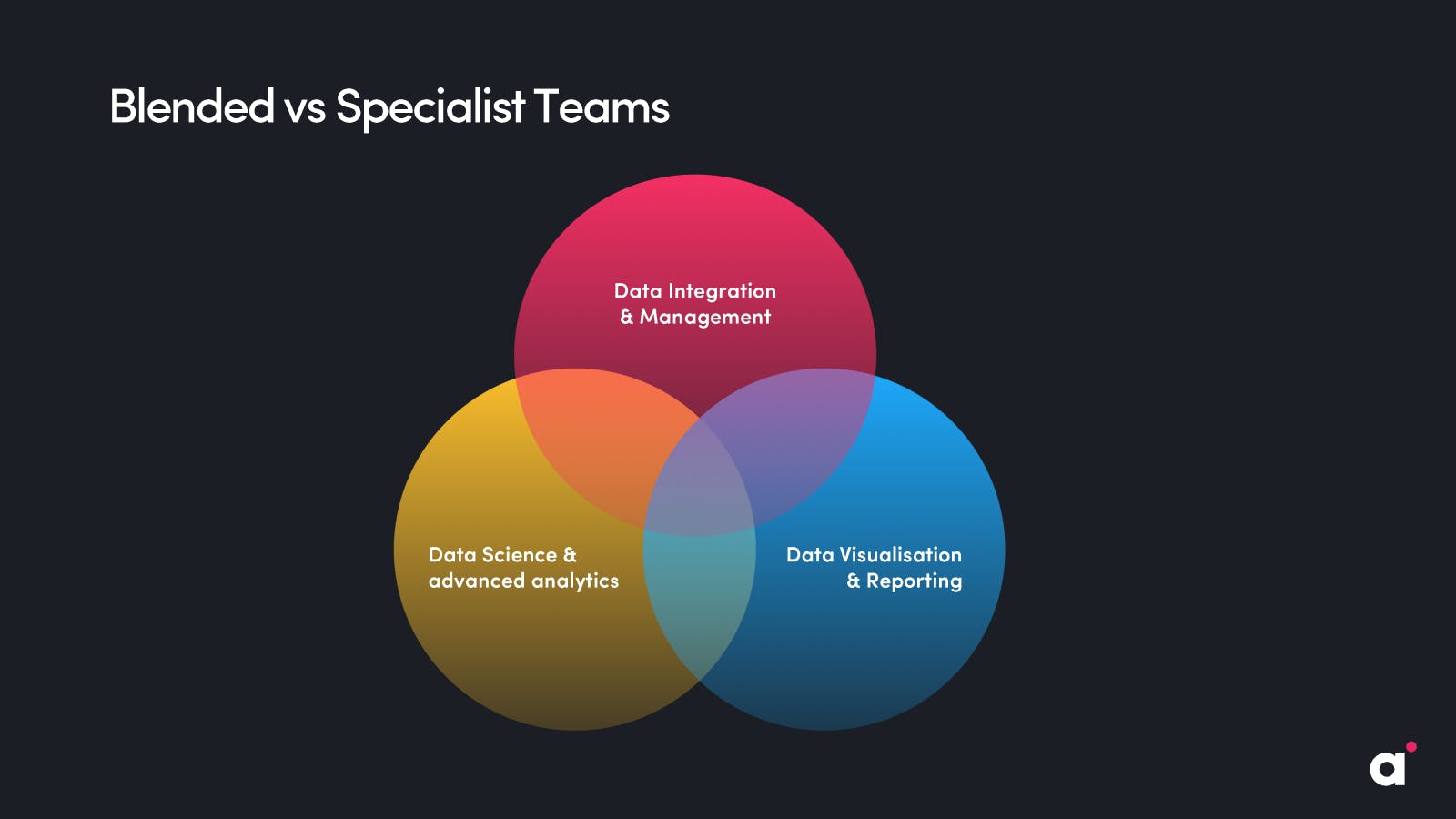
If you are looking to setup a specialist data science team then look out for our free data science roadmap template coming soon.
Define data (user) personas
You’re probably thinking “Personas, isn’t that a UX thing?”. Yes, but it also works well in a data context. Over the years I’ve heard – and used – several metaphors to explain how users interact with data. I think my favourite one has got to be ‘surfer, swimmer, diver’ – I’ll let you try and work that one out. Rather than confuse everyone with metaphors, data personas say it like it is – for each type of user, a persona tells us who they are and how they are going to use data. In many meetings, these personas have helped the business relate to the data team function, as well as showcasing that the data team has considered the various roles and needs of the business.
When defining your personas, make sure to include all roles that use and interact with data. This includes members of the data team itself. Here’s an example of setting up user personas for a data team.
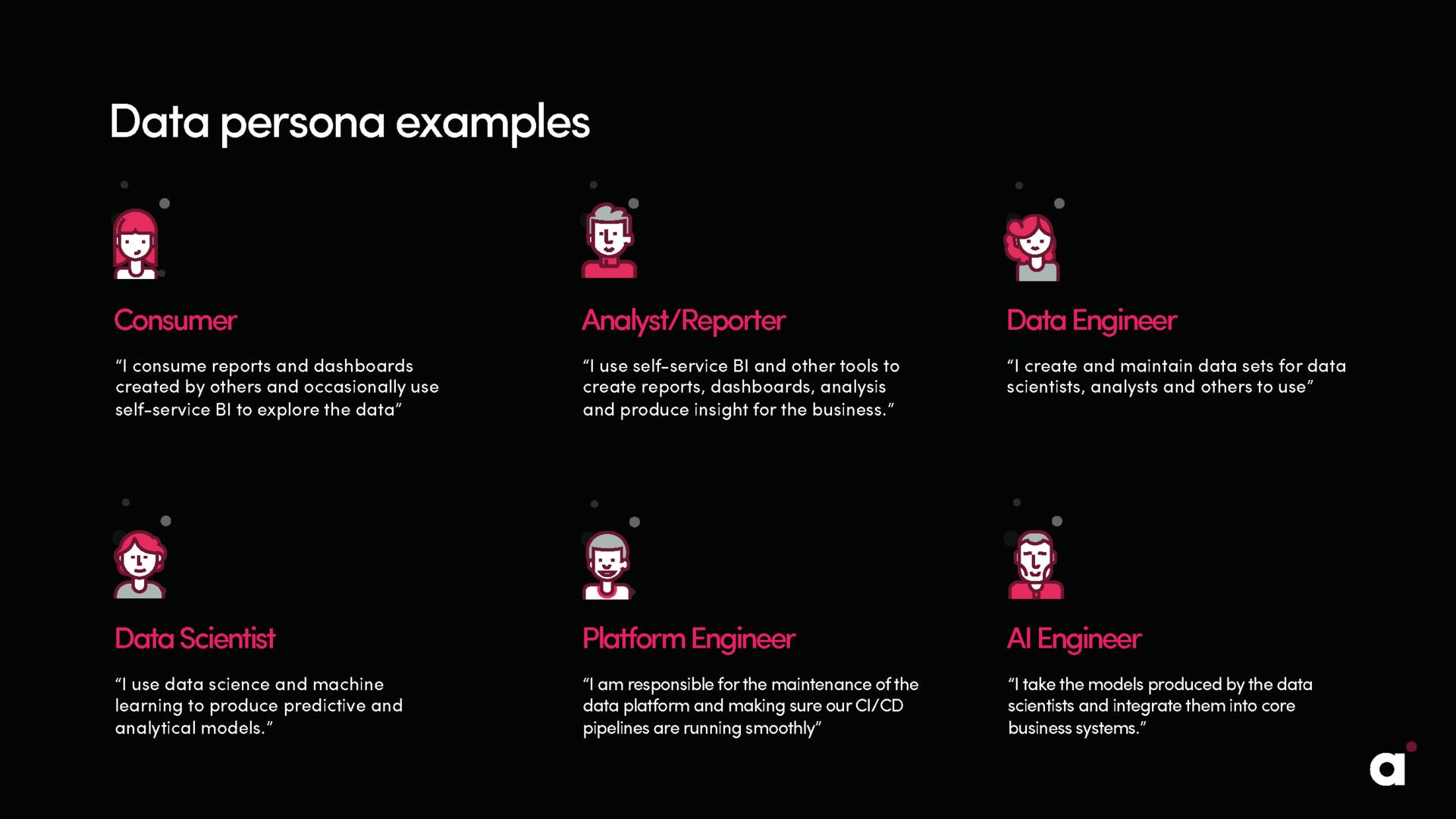
Build a list of data products
Whether you’ve got an existing list of data requests, or you’re looking to set up a new list of these for 2020, you need to have a pipeline of work and projects that are going to deliver against the vision. These will contribute to your initial set of data products, which you’ll continue to enhance and develop. One of the most important things when building this list is to make sure you capture the business value – this could be in operational savings, risk reduction, new revenue or the influence on a performance metric of the business. Doing this will help both prioritise and measure the success of these data products. More on this later.
Update your data platform
With renewed vision comes the need for new functionality. Whether you have decided to go with a blended team or need to work in conjunction with other specialist teams, your data platform needs to support this. The functionality of ingesting data from source systems and storing it in a centralised data store (be it a data lake or a data warehouse), and the ability to surface this data to front-end tools through semantic and analytical models, is the standard that most platforms provide.
If your vision includes data science or AI, then providing an environment for data scientists to build, test and productionise models is crucial. As is supporting the integration of AI apps into front-end systems. In some cases, you may need to revisit your architecture, whilst in other more modern (cloud-based) platforms it could be as simple as enabling new components within the existing platform.
Get comfortable with Unstructured Data
Data teams have been extracting data from disparate data sources for years now. They expect to have an underlying database from which they can extract data, and this works well for when you have a front-end application based on a standardised process. However, when faced with the challenge of bringing in data from an offline process which is document or content based, data teams are often stumped. This becomes even more challenging when you have to deal with unstructured data from email or social media. Ensure the data platform you choose supports unstructured data, so that you can start to address this challenge. And when analysing potential data sources to solve a problem, make sure you don’t rule out content, document or email data sources.
If you want to learn more about tapping into the hidden value of unstructured data sources, read about Aiimi’s Insight Maker platform here.
Implement a DataOps approach
To support the speed at which the business is expecting results, the approach to building and deploying the data products I mentioned earlier will need to change. This is where DataOps comes in. If you haven’t already heard about DataOps, this gives a good overview.
DataOps focusses on continuous integration and continuous development, with a view to reducing the time to market for your data products. Simply put, this means building in an agile manner, applying DevOps processes to data operations and maintaining a focus on people and collaboration.
DataOps is too broad a topic to cover in this article so look out for an article by my colleague Paul Sliwinski on a practical approach to data ops. Coming soon!
Measure the success of data products and the team
Earlier in this post, I mentioned capturing the business value that a data product will add – if it’s implemented successfully. Time to market, quality and take up are all factors that will determine whether it creates business value or not. It’s worth bearing in mind that, even if it does not generate the intended business value, your product is not entirely a failure. Due to the shorter development cycles, you can afford to fail. The objective is to iteratively improve the quality of your data products.
Standard performance measures of BI teams (such as response or resolution times) still hold good, but DataOps comes with its own set of performance metrics. Very often these can get massively detailed and complex, and in some cases they need to be. But, in most cases, answering the four following simple questions should give you all you need to know:
- How much value are we adding to the business?
- Are we responding to customers on time?
- Are we finishing work when we said we would?
- Are our customers (end-users) happy?
- Is the team happy?
- Can we do better?
This is an exciting time for data professionals and leaders; the appetite for better quality, faster and smarter data is constantly growing. Some BI teams will be able to cope with this demand with little or no change, but others will redefine the way they operate and reinvent themselves as blended or specialist data teams. The above list of recommendations is a snapshot of the data journey which I was part of, hopefully it will help with yours. All the best.
Aiimi Insights, delivered to you.
Discover the latest data and AI insights, opinions, and news from our experts. Subscribe now to get Aiimi Insights delivered direct to your inbox each month.
Aiimi may contact you with other communications if we believe that it is legitimate to do so. You may unsubscribe from these communications at any time. For information about our commitment to protecting your information, please review our Privacy Policy.
Enjoyed this insight? Share the post with your network.
What are Data Sprints and how do they work?

Data Sprints – why run a data project when you can sprint

How to apply AI for Business Intelligence (an ideal first GenAI use case)

The benefits of data governance in the age of AI: delivering trust, supporting innovation

The rise (and risks) of Edge AI
