ChatGPT Explained: A breakdown of how it works for curious business leaders.
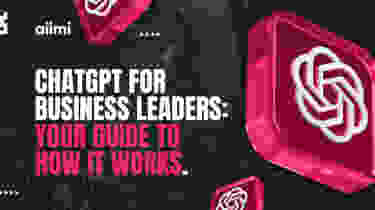
There’s a lot of buzz about AI at the moment, much of it prompted by the launch of ChatGPT at the end of last year. ChatGPT has brought AI into the public domain, making it possible for anyone to use AI to generate content. (More about generative AI and how it works below). For the first time, it’s not just tech companies, business leaders and politicians talking about AI, it’s lawyers, teachers, writers and the mainstream media, too. Many people are excited about the potential of ChatGPT for business efficiency, the time it will save on researching and drafting content, and the speed at which vast amounts of information can be analysed. Others are worried about its ethical challenges, opportunity for misuse, and data security concerns when used by employees.
While there are other chatbots out there that leverage generative AI, ChatGPT is the most widely used at the moment. (It had 100 million users within 2 months of launch, compared to TikTok, which took 9 months to reach this milestone and Instagram which took 2 years. Only recently has this record been surpassed by the launch of Meta’s Threads platform). In this blog, we’ll go back to basics, breaking down some of the concepts behind ChatGPT and the Large Language Models that this kind of AI is built on – and what this means for business adoption of AI.
What kind of AI does ChatGPT use? How does it fit into the AI landscape?
First things first, let’s unpack some of the ways AI already works all around us.
AI is not new. ChatGPT has created a phenomenal buzz, but we’ve all been using AI to extract, generate, and predict for a long time now. It powers digital assistants, such as Amazon Alexa, Siri, and Google Assistant in our homes and on our smartphones, and it drives the helpful tech that predicts what you might want to say in emails and texts.
In the business world, AI chatbots have taken over customer service, and some form of AI underpins the way most medium to large-scale retailers, streaming services, social media, banks, and more, use data to predict customer needs, protect sensitive or personal information and secure transactions.
All these examples make use of several AI technologies, generative AI included, which are driven by their ability to understand human language. Natural Language Processing (NLP) sits at the heart of many of these AI applications and enables them to respond to prompts from users in all kinds of contexts in the home or the workplace. NLP gives computers the ability to understand text and spoken words – not just read them but understand their meaning and intent. A good example of this can be seen in our basic interactions with digital assistants like Siri or Alexa – a user prompts the assistant to “Turn down the volume”, in their own natural language, AI understands the intent, and a response or action is triggered. Beyond this, NLP provides the ability to respond in natural language too, which comes to the fore in use cases such as chatbots.
How does ChatGPT generate answers? What makes ChatGPT different?
Generative AI itself isn’t new, and there are also lots of exciting developments in other areas of AI, too. So, what makes ChatGPT special? An easy way to explain how it works is to ask ChatGPT itself. This is how it replied:
ChatGPT is an advanced language model developed by OpenAI. It utilizes the Generative Pre-trained Transformer (GPT) architecture to enable human-like conversational interactions. Trained on a massive amount of text data, ChatGPT can understand and generate coherent responses to user queries. It leverages its extensive knowledge base and language understanding capabilities to provide information, engage in creative writing, and offer suggestions across various topics and domains.
A pretty comprehensive response that sounds clever, and impressive – and it took only seconds to generate. There’s one key theme that comes out from this AI-generated response, and that’s ‘scale’. Trained on a massive data set (the internet), ChatGPT has developed a vast knowledge base and a remarkably human-like ability to understand and generate natural language. Other generative AI tools based on Large Language Models (LLMs) simply haven’t been developed at this scale, and the quality, range, and naturality of the results they deliver are rather more limited and less convincing.
But one important point that ChatGPT has missed from its self-generated autobiography is how it’s been trained. OpenAI’s training process uses Reinforcement Learning from Human Feedback (RLHF), a technique which involves human trainers providing feedback to the AI model, helping it establish common preferences which influence its future outputs. This is one of the major factors that’s helped ChatGPT become a game changer in generative AI.
How accurate is ChatGPT?
With a vast data set like the internet, bad data comes along with the good data.
Every ChatGPT answer comes with the caveat that “ChatGPT may produce inaccurate information about people, places, or facts”. Like any AI system, ChatGPT is only as good as the data it has been trained on – and the engineering that’s been done to make sure bad data doesn’t sway its outputs. With the internet, there’s an inevitable level of inaccurate data or data containing unconscious or overt bias such as racism and sexism. RLHF and other guardrails have been used to help ChatGPT favour certain ways of responding, or avoid troublesome topics entirely, but users have found ways to bypass these measures and churn out some undesirable results.
Generative AI also has a tendency to “hallucinate” in certain scenarios, i.e. make up information and make it sound real. A naive US lawyer used ChatGPT to speed up his legal research, but it generated completely false case studies, which cost him the case, and his reputation.
These hallucinations, inaccuracies, and bias can appear because generative AI is probabilistic. At its most basic level, an LLM learns to predict the next word or sequence of words depending on the input. There are always several options, meaning it can generate and regenerate many different answers to the exact same input. With its extensive fine-tuning and RLHF, GPT-4 (the LLM that powers the ChatGPT app) is more advanced than any other LLM in its ability to apply reasoning and solve problems – that’s what makes it so impressive. But potentially inaccurate or biased information brings obvious problems for any enterprise that uses this technology without understanding its risks and limitations – from basing business decisions on flawed data to damaging their reputation and integrity when AI-driven mistakes come to light. The potential for hallucinations and inaccuracies highlight why human validation and careful consideration of appropriate use cases are so important when using generative AI for business.
What are the main issues with generative AI for business?
Let’s summarise some of the main concerns that arise when considering using ChatGPT or other generative AI tools in the enterprise.
1. Inaccuracy and bias
Any AI is only as useful as the data it has been trained on, alongside a few other factors. ChatGPT, for example, does not have access to real-time information. In fact, it does not contain any data post September 2021. Whilst this is perhaps less relevant in formal business use cases, where you’re unlikely to be using a public model like ChatGPT, this limitation should not to be overlooked when considering how your employees might be using ChatGPT to enhance their day-to-day work. This could lead to instances of introducing bad code, basing research or content on outdated information and even internalising bias or misinformation.
Fine-tuning LLMs with high-quality, up-to-date data and using enterprise search solutions to enhance user prompts and expose the model to the most relevant information, are both excellent ways to reduce the risks of inaccuracy in enterprise use cases for generative AI.
2. Data security
Data security and protection, as well as compliance with data and privacy regulations, can also present problems. No one really knows how data is used once it's shared with public AI models, which is extremely concerning. If employees have unrestricted use of ChatGPT, it can lead to businesses no longer being in full control of their own and their customers’ (often sensitive) data. Similarly, it can also be hard to protect intellectual property. If employees are copying data and sending it to ChatGPT, or other public LLMs, you can no longer control what happens to it.
Even within private LLMs, internal data security can be an issue. If models are supplied with enterprise data, you need to ensure that only those with permission to access the source data can see the output – or risk employees stumbling across information they should never have been able to access.
3. Ethics & Individuality
Ethically, generative AI can also be a challenge – it’s a powerful tool which can certainly be used to do harm, as well as good. Claims of plagiarism and cheating, particularly in academic circles, are regularly levelled at ChatGPT, and we have no real way of fully comprehending the ethical biases which may be present in training data used in LLMs. Plus, the art of ‘prompt engineering’ is a whole new field of expertise and development, emphasising the importance of how we ask, relative to the answers we get.
On a related note, although ChatGPT can generate text in a range of styles and in a fraction of the time it takes a human, there is a danger of a business losing its unique voice and personality if it relies too heavily on AI-generated content.
Should we use ChatGPT for business at all? Are there broader uses of Generative AI for business?
A lot of businesses will be tempted to jump quickly on to the ChatGPT bandwagon. However, at Aiimi we’re adopting a more cautious and considered approach to using any public LLM as well as to the potential business applications for generative AI. ChatGPT is phenomenal and has a wide range of possible uses, but it is a public model with serious limitations. We do not recommend using it with any sensitive corporate data or integrating it into any existing software tools. Read our CEO’s thoughts on this here.
The broader potential of generative AI technology is hugely exciting, but the pitfalls for those who get it wrong could be catastrophic. At Aiimi, we know the importance of trustworthy data and its value to organisations, so quality data engineering – built on a foundation of getting the complete picture of your data – and due diligence in where we apply models will be at the core of everything we do with generative AI.
For us, setting the context and purpose of what organisations want to achieve with AI is vital. How do we want AI to help us? What do we need it to do for us? In the first instance, this is likely to mean fine-tuning private models with specific data sets. If we can trust the data that goes into the model, then we can more readily trust the information that comes out. LLMs can help us push beyond the limits of just extracting information, but extractive AI models can still have a significant role to play, too. Sometimes, all we need AI to do is crunch through a huge set of documents, pull out key information for us, and tell us where it came from.
So, is the hype about ChatGPT and generative AI for business completely unjustified?
Not at all! Generative AI is, according to Sam Altman, CEO of OpenAI the creators of ChatGPT, potentially going to have the “biggest impact on creative workflows since the computer itself” – and the opportunities that such mainstream coverage opens up for this technology are huge. But it comes with many risks, limitations, and a whole lot we don’t yet know. In business especially, leaders need to advocate using AI technology responsibly and carefully, considering exactly what business needs it can serve and ensuring the quality of the data the AI is trained on. And we need to be absolutely sure that we can continue to control how our corporate data is managed, used and shared.
Stay in the know with updates, articles, and events from Aiimi.
Discover more from Aiimi - we’ll keep you updated with our latest thought leadership, product news, and research reports, direct to your inbox.
Aiimi may contact you with other communications if we believe that it is legitimate to do so. You may unsubscribe from these communications at any time. For information about our commitment to protecting your information, please review our Privacy Policy.
Enjoyed this insight? Share the post with your network.
How to apply AI for Business Intelligence (an ideal first GenAI use case)

Llama 2: Our thoughts on the ground-breaking new large language model

Top-priority GenAI use cases for your business

How to apply AI for Business Intelligence (an ideal first GenAI use case)

The benefits of data governance in the age of AI: delivering trust, supporting innovation
