Why I’m advising against using ChatGPT for business – and what to do instead.
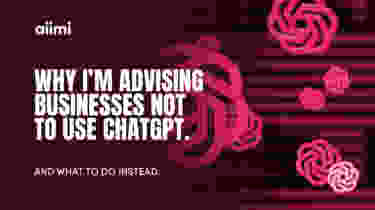
“Can ChatGPT help solve some of our biggest business challenges, and fast?”
“Isn’t Generative AI a new tech frontier that’s going to make ‘the old ways’ redundant?”
“It looks like everyone’s using ChatGPT for business; surely we need to get on board now?”
If I had a pound for every time an Aiimi customer or prospect has asked me one (or more) of these questions in recent months, I’d have accumulated quite a sizeable savings pot – though admittedly not enough to train a Large Language Model like OpenAI’s GPT-3. (Experts in the field estimate you’d need over $4 million to do that, but more on why the staggering costs of training LLMs matter later…)
The rise of ChatGPT for business
The interest in ChatGPT for business and individuals isn’t a surprise. It’s been touted as “the best artificial intelligence chatbot ever released to the general public”, garnering coverage from technologists across the globe. This is the first time we have seen an AI algorithm apply extensive general ‘knowledge’ to solve problems in natural language processing models – based on quite complex demands like writing code, explaining difficult topics in layman's terms, or even drafting poetry.
Couple this with an impressive conversational user experience and learning reinforced by human feedback, ChatGPT’s users have been left wondering, “How does it generate content?” time and time again. With results that are faster and (often) more accurate than a human could ever produce, the mass hype for ChatGPT is understandable.
Outside of all the media excitement, I’m pleased that our customers and personal contacts are coming to Aiimi for expertise and guidance on using Generative AI for business. That’s why we’re here – to help organisations use data and AI technology to solve big challenges and secure competitive advantage. And that doesn’t always mean the most newsworthy Machine Learning techniques. Established approaches like Extractive AI may be more useful in near term use cases than their shinier, newer counterparts.
Generative AI: is it safe?
Still, Generative AI can potentially deliver fantastic results for enterprises. Coupled with the linguistic capabilities of well-trained models, the technology can create flexible summaries, answer questions, and spin brand-new content – everything from customer insights to new product designs. I’m genuinely excited about what Generative AI can do for businesses; not least because it’s accelerated business leaders’ understanding of and ambitions for AI technology at all levels.
But Generative AI is fallible – Large Language Models are only as good as the data you put in to train them. Plus, they have tendencies to ‘hallucinate’, giving convincingly natural answers that are completely inaccurate. As with all new technologies, we’re on the rollercoaster of a hype cycle with Generative AI – and that means we’re inevitably going to see fallout before the landscape levels and practical enterprise use cases become apparent.
With ChatGPT – the most public and popular example of Generative AI right now, fallibility, privacy, and security concerns are rife. But that hasn’t stopped tech providers from building enterprise integrations, or their customers from adopting them at speed.
How are we directing our Aiimi customers to use ChatGPT?
We’re advising them not to.
For most organisations, I believe the best approach right now is to wait – to not use any of your corporate data with ChatGPT. That means advising your employees what they can and can’t use the ChatGPT web tool for, and potentially even restricting access via corporate devices. And it definitely means saying “No” to some of the ChatGPT integrations that are springing up in your existing enterprise tools, from official product releases by Microsoft Azure and Shopify, to developer-led solutions in ServiceNow.
“For most organisations, I believe the best approach right now is to wait – to not use any of your corporate data with ChatGPT.”
With the ChatGPT API, integrating the technology with your existing tools isn’t technically difficult. Like many others, we’ve tested it (securely) with our own Aiimi Insight Engine platform – using ChatGPT to provide Q&A and summarisation capabilities for specified data sets, curated by running an initial search using our platform. This is the base capability that so many enterprise tech providers are demoing and rolling out as a new feature for their customers.
What does ChatGPT do with your enterprise data?
The simple truth is we don’t know what happens to enterprise data when it’s sent to Large Language Models like ChatGPT. The model’s creators haven’t made this clear. We don’t know whether the data you put in is fed back into their training model, potentially being used to generate results for other users down the line.
Even if companies like OpenAI did state that they don’t use corporate data for training, would you fully trust this claim? Most organisations would never risk losing IP or breaching privacy promises they’ve made to their customers, employees, and partners.
And when it comes to the accuracy of the results you get back when you ask ChatGPT to work with your enterprise data out of context, who knows whether the answer to your query will be relevant or correct, even if it looks convincing. After all, the model has only been trained on internet data. It has no idea what the world looks like from the perspective of your organisation. It has no access to your complete data universe.
Protect your data and IP at all costs
At Aiimi, we’ve chosen not to offer a ChatGPT integration to our Insight Engine customers for the time being. It comes down to protecting your data and IP – and having confidence that ChatGPT can create usable results from any data you give it. Without those two big challenges clearly resolved, the risks of commercial instability, data loss, and poor decision-making far outweigh the benefit of trying something new.
I understand why some business leaders will be more than a little disappointed to realise that ChatGPT isn’t the silver bullet to crack enterprise-scale LLMs – but it’s not the end of the road for the business applications of Generative AI right now either. There are plenty of other options for using Generative AI securely – not least other LLMs.
“Some business leaders will be more than a little disappointed to realise that ChatGPT isn’t the silver bullet to crack enterprise-scale LLMs – but it’s not the end of the road…”
So, instead of building a ChatGPT integration for Aiimi Insight Engine, we’re helping our customers realise results from smaller, private (and therefore secure) Large Language Models. These represent the biggest tangible opportunities for organisations right now – and avoid the major risks of using public models like ChatGPT with your private data. By taking an LLM and fine-tuning it with enterprise data to create a private, internal model, you can improve the data quality and remove the risks that make ChatGPT potentially unsafe, irrelevant, and inaccurate.
Size also matters when it comes to language models. Training a large private model from scratch with your entire enterprise data set could cost a substantial amount (training GPT-4 cost “more than $100 million”, according to OpenAI CEO Sam Altman) and take days or weeks to complete, making real-time access to data insights impossible.
Focusing on using smaller, curated datasets to fine-tune existing LLMs with clearly defined use cases in mind is a practical solution. Plus, with smaller data sets, you can expect relevant, accurate results, as it's feasible to keep the model bang up-to-date and trained on your latest data.
Get to your answer with Extractive AI too
Take an example of using Generative AI to help design a new vehicle – imagine feeding a model with datasets related to your products, everything from CAD designs and car telemetry data to customer feedback. This is highly sensitive commercial IP. It can’t leave the enterprise – and you need to be confident any complex new recommendations your model generates will pass muster when it comes to product requirements, like regulatory compliance, or health and safety.
There’s no room for unchecked hallucination or inaccuracy in these use cases, as results not grounded are not only useless, but potentially catastrophic. This is where bringing in Extractive AI, a well-established technology in enterprise platforms like insight engines, can be very useful, especially when citation is key to realising usable results.
With Extractive AI, we can cite specific documents and data sources, which makes it easier for the user to know where the answers are coming from. Building capabilities that can leverage both Generative and Extractive AI technologies is essential for many enterprise use cases.
With Generative AI, a robust training data set is necessary to get usable, reliable results – and while citation can be done, it’s nowhere near as precise as with Extractive techniques. This is why we’re pushing our customers to focus on data quality and data engineering first. With any data model, the old computer science adage remains true - garbage in, garbage out. Using high-quality corporate data sets for privately fine-tuning Large Language Models will help ensure you get more accurate, relevant results.
“Using high-quality corporate data sets for privately fine-tuning Large Language Models will help ensure you get more accurate, relevant results.”
To deliver these high-quality training datasets at speed, you need technology that can discover all relevant data, structured and unstructured, no matter where it lives in your organisation, and automatically enrich it to guarantee data quality, ensure security, and access controls all the way through to end-use.
When you know exactly what you’ve put in, the limits of this data, the tests, and quality checks you’ve run upfront, and the cross-checking you’re able to do once the model is live, only then can you reach the level of assurance you need to practically use Generative AI technology in your business.
Data quality also means having a complete, representative data set. I’m cautious about technology providers limiting their model creation capabilities to their own stacks. The average enterprise has over 350 systems and applications, from a wide range of providers. While integrated, private model-building capabilities may be useful additions to these systems, they likely won’t be able to bring in data from across the enterprise – a crucial step in getting the most relevant, accurate training data that’s needed for powerful results.
Invest in private Generative AI models
My guidance, and the advice we’re sharing at Aiimi, is to take the high road. Don’t fall foul of IP loss, security breaches, data privacy challenges, or the consequences of basing business decisions on inaccurate results. Putting your enterprise data into public LLMs like ChatGPT may offer some short-term gain and novelty, but we don’t know what the long-term consequences will be.
Invest in fine-tuning private models to bring the power of Generative AI into your enterprise and exercise control over your data. But leave public models like ChatGPT at the door.
Explore more practical advice, success stories, and expert insights. Visit the Aiimi CIO+ Hub.
Aiimi Insights, delivered to you.
Discover the latest data and AI insights, opinions, and news from our experts. Subscribe now to get Aiimi Insights delivered direct to your inbox each month.
Aiimi may contact you with other communications if we believe that it is legitimate to do so. You may unsubscribe from these communications at any time. For information about our commitment to protecting your information, please review our Privacy Policy.
Enjoyed this insight? Share the post with your network.
The definition of insight

The rise (and risks) of Edge AI

How to apply AI for Business Intelligence (an ideal first GenAI use case)

The benefits of data governance in the age of AI: delivering trust, supporting innovation

The rise (and risks) of Edge AI
