How to improve customer experience with data analysis.
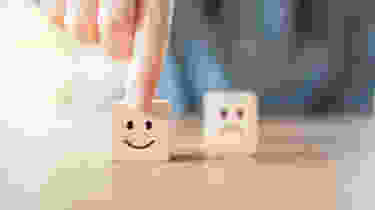
The customer forms an integral part of almost any business. Any organisation offering a product, service or experience requires someone to purchase their wares. And for a customer to keep on purchasing goods from you, you must keep them satisfied! The motivations behind achieving customer satisfaction can vary from industry to industry; sometimes you must differentiate yourself from or outperform a competitor, other times you must maintain a level of service or risk punishment from regulators. If you’re reading this article, I’m sure you already know all of that – what you really want to read about is data, and how you can use yours to make achieving customer satisfaction even easier.
I want to draw on two ways of working with customer personas – one a more traditional, journey-focussed approach to identifying personas, and one that uses data to take a deeper dive into the customer journey. Let’s see why taking that closer look with data can help you improve your customers’ experiences…
The traditional approach: Identifying personas using customer journeys
Traditionally, you can identify customers by examining the journeys that they take in their contact with you and the interaction points that they touch, sometimes inadvertently. The more seamless and personal the journey that you can offer your customer, the more satisfied you’re likely to make them.
A mobile application is a great example of this. Apps can be simple and clean to use for many customers, but what if a customer doesn’t own an appropriate device? One simple element of the journey has already divided our customers into two distinctly identifiable segments – those who can complete it using the app, and those who can’t.
By applying this kind of thought process to customer journeys we can begin to identify personas based on other factors too, like age, gender, location, profession, education and technology used.
However, by using these as criteria to segment customers, it is likely that you’ll carry forward some element of unconscious bias and pre-defined misconceptions into your personas.
The alternative approach: Understanding personas using data
So, how else can we segment and understand our customers, other than by using the basic demographic and technological information that we capture about them at their interaction points?
The data that we capture about customer journeys can be so much better at telling us the real story. We can use data to segment happy customers from dissatisfied customers. Even more importantly, we can identify the parts of the customer journey or the operational features that are contributing to negative experiences.
Often, it is not a lack of understanding or incorrect profiling of a customer that is leading to their dissatisfaction, but rather a poorly performing element of the service you’ve provided. It’s crucial to be able to recognise problems like this if you want to make improvements.
By analysing the data from real customers’ journeys, along with known outcomes from previous interactions and customers journeys, you can begin to identify those who are experiencing negative outcomes. The crux of this is that, rather than simply analysing the characteristics of a customer persona, you’re also analysing the experience that they are provided by your product or service.
Applying analytics to improve the customer experience
Taking this a step further, how can we use this data about customer experiences to improve customer satisfaction? This is where you don’t just want to be reactive in your use of analytics. Building a predictive model for the outcomes of your customers’ journeys enables you to identify what a customer might be likely to experience at various points along that journey. Where these predictions differs from your expectations, or from the reality that a customer is experiencing, you know you’ve got an ideal area to make improvements.
Furthermore, using real data to understand what customers are experiencing on their journey, rather than making assumptions based on the information we know about them, reduces the effects of human bias and pre-conceptions and lets the truth do the talking.
How to improve customer satisfaction in the water industry
Here’s an example of the process I’ve just described in action in the water industry…
Water companies hold data including customer information records (in a CRM system), customer billing data, complaints data, and data about specific jobs and operations they’ve conducted. By piecing together job data and customer data, we can get a much more complete picture of what a customer’s experience is like – from their first call to report a problem, to the operative logging this in the system, to sending out a repair team, to the arrival and departure times of that team.
By doing this, we can start to identify points in that customer experience which can be associated with a particular outcome. And by doing this across a whole set of historical data about jobs and customers together, you can begin to see the scenarios which repeatedly lead to a positive result – and those which don’t.
It might sound obvious that if the service team arrives on time to a job, the customer will be much more likely to leave a positive review of the company, but some results from data analysis like this will be more unexpected. For instance, let’s say the team arrives on time to a job (meeting the timeframe defined in their SLA, Service Level Agreement), but the customer still leaves a negative review. Why might this be? Perhaps the problem is so dire that resolving it will never turn it into a positive experience, no matter how effective or efficient that resolution is; or maybe the SLA timeframe that’s in place for this type of job needs reassessing.
By identifying the subset of features from every job which consistently lead to an outcome, whether positive or negative, we can then begin to investigate which features (or combinations of features) are representative of a particular outcome. Using historical data, you can predict what outcome you will likely get from a known scenario. Then, you can use this to test out new scenarios and establish the likely outcome from these too.
What’s the point of all this in improving customer experiences? Well, now you can start to identify those customers and scenarios which are more likely to result in a negative outcome and work proactively to turn those customer experiences into positive ones.
Here’s what data analysis can actually do for customer experience
Still, there are some limitations to what we can understand with data analysis. For instance, customers who always give negative feedback with no apparent reason, or customers who give positive results regardless of the outcome – data analysis can’t get to grips with these ‘illogical’ choices.
What analysis can do though is to pinpoint and help organisations to address the scenarios and customers whose outcomes can be swayed; whose score will change based on the experience they're provided.
Imagine the following: David has a burst pipe in his garden, and your customer service team promises to be there on Tuesday. But on Tuesday morning you’re alerted to a bigger job – a burst water main at a school – and you can’t make it to David’s property. A quick courtesy call to inform David that your team will be over later that day could be the crucial factor in ensuring that David’s feedback is much more positive. But how do you know exactly which action will have the most impact? This insight is what the data analysis I’ve described can give you.
In the new world of C-MeX (Customer Measure of Experience) for the water industry, customers score their experiences on a scale of 0 to 10, so it is vital to improve those experiences. Customers who give a score of 4, for instance, may have had a slightly negative experience which could be very easily improved, likely transforming them into a more satisfied customer with a score of 5 or above.
Data analysis can pinpoint the exact actions or features of scenarios that can make this difference – and which customers and scenarios are most likely to have their experience transformed from negative to positive if these actions are included. Don’t make assumptions – figure out exactly the most important actions to take, and what the cost of implementing them is, rather than just rolling out new actions (like a courtesy call when you’re running a bit late) at scale.
How to make the most of your data model
Of course, data alone will not solve the problem of understanding why customers have the experience they do – context is always important when looking at results. For example, uncommon outcomes are not particularly well served by this kind of data analysis. Why? Because the journey and experiences involved are not covered across the rest of your data set. If data about this outcome isn’t already in existence, then a predictive model won’t know how to handle it. It is not that the model is wrong, it simply doesn’t have enough information to know – a little like us sometimes!
The more data you can feed your model with, the better and more accurate its insights will be.
When data is combined with human domain knowledge and is given the appropriate value in your analysis, you can understand so much more about the products and services that you provide. No matter how you go about it, understanding this is the key to ensuring that you provide the best experience for all your customers, always.
Using data analytics and machine learning, the Aiimi team has helped several of our customers in heavily regulated industries to better understand the experiences that they provide to their customers. This has helped them to identify the causes of negative outcomes and better retain and satisfy their consumers. Find out more about how our Data & Analytics services can amplify your insight.
Aiimi Insights, delivered to you.
Discover the latest data and AI insights, opinions, and news from our experts. Subscribe now to get Aiimi Insights delivered direct to your inbox each month.
Aiimi may contact you with other communications if we believe that it is legitimate to do so. You may unsubscribe from these communications at any time. For information about our commitment to protecting your information, please review our Privacy Policy.
Enjoyed this insight? Share the post with your network.