Asset management in the age of AI: Unlocking insight across the asset lifecycle.
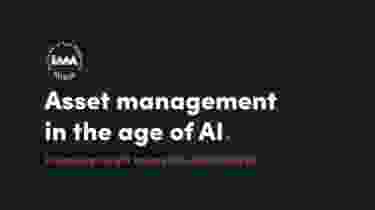
Missed our recent 4-part webinar series on Asset Management in age of AI, developed in partnership with Institute of Asset Management? Check out the boxset of all four bitesize sessions in the video playlist below, or read Helen Patenall's summary of the highlights.
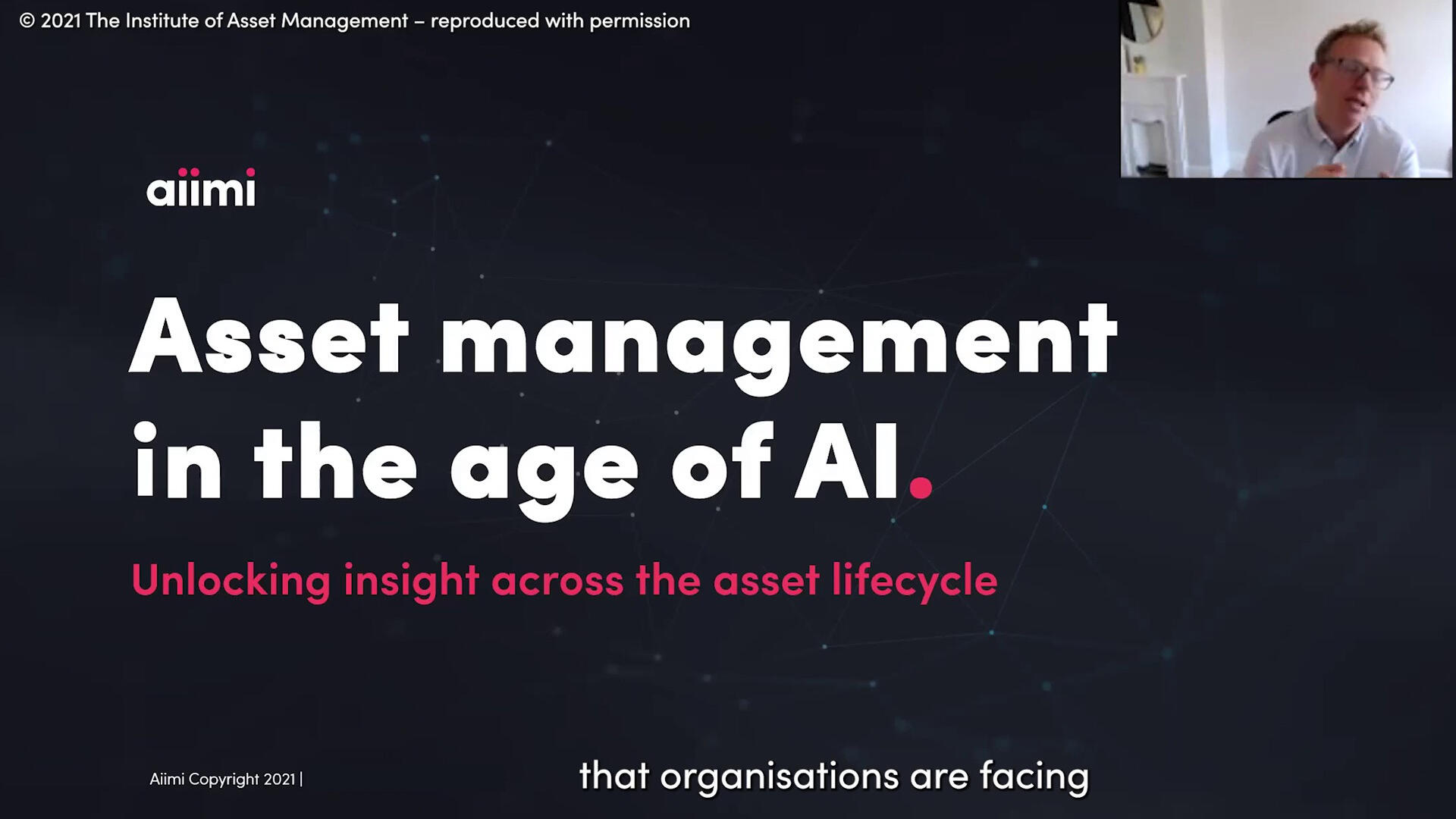
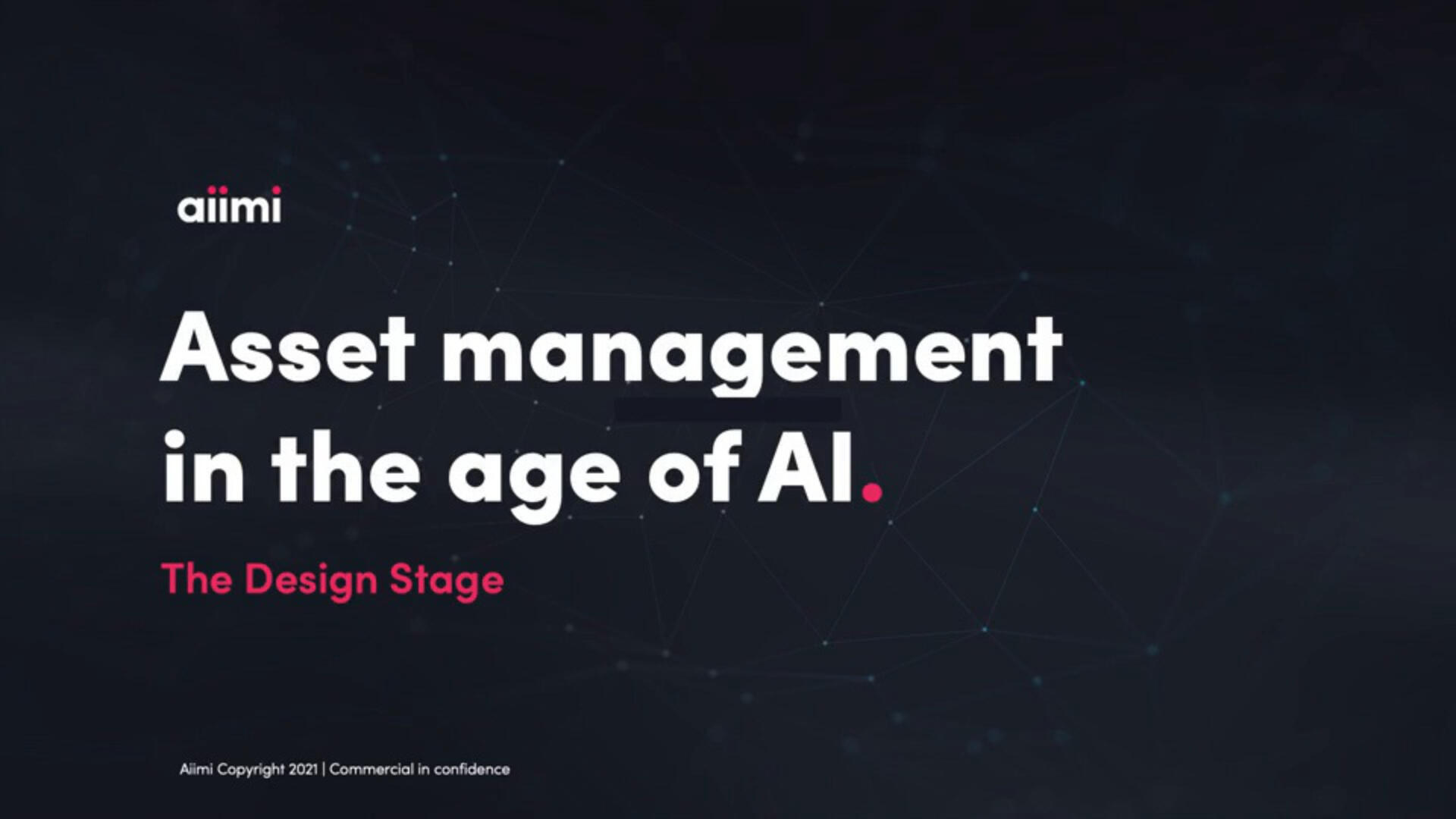
Unlocking insight across the asset lifecycle – three founding principles
As more data is created, our ability to separate the wheat from the chaff – and join the dots between critical data – gets harder. Rather than adopting a centralised data repository system to tackle these underlying challenges, savvy asset-centric organisations are embracing an entirely different approach. They’re taking great strides in adopting AI technology and machine learning to capture high-quality data and information across their asset lifecycle, and make it readily available to all their users. By directly connecting their asset teams to all that interconnected data, they’re also unlocking meaningful insight, realising its full potential value.
So how should asset-centric organisations go about replicating this successful approach to asset management? The first step is to incorporate three core principles when dealing with data and information across every stage of your asset lifecycle.
- Treat data and information as equal partners
- Automate information governance
- Focus on the user experience
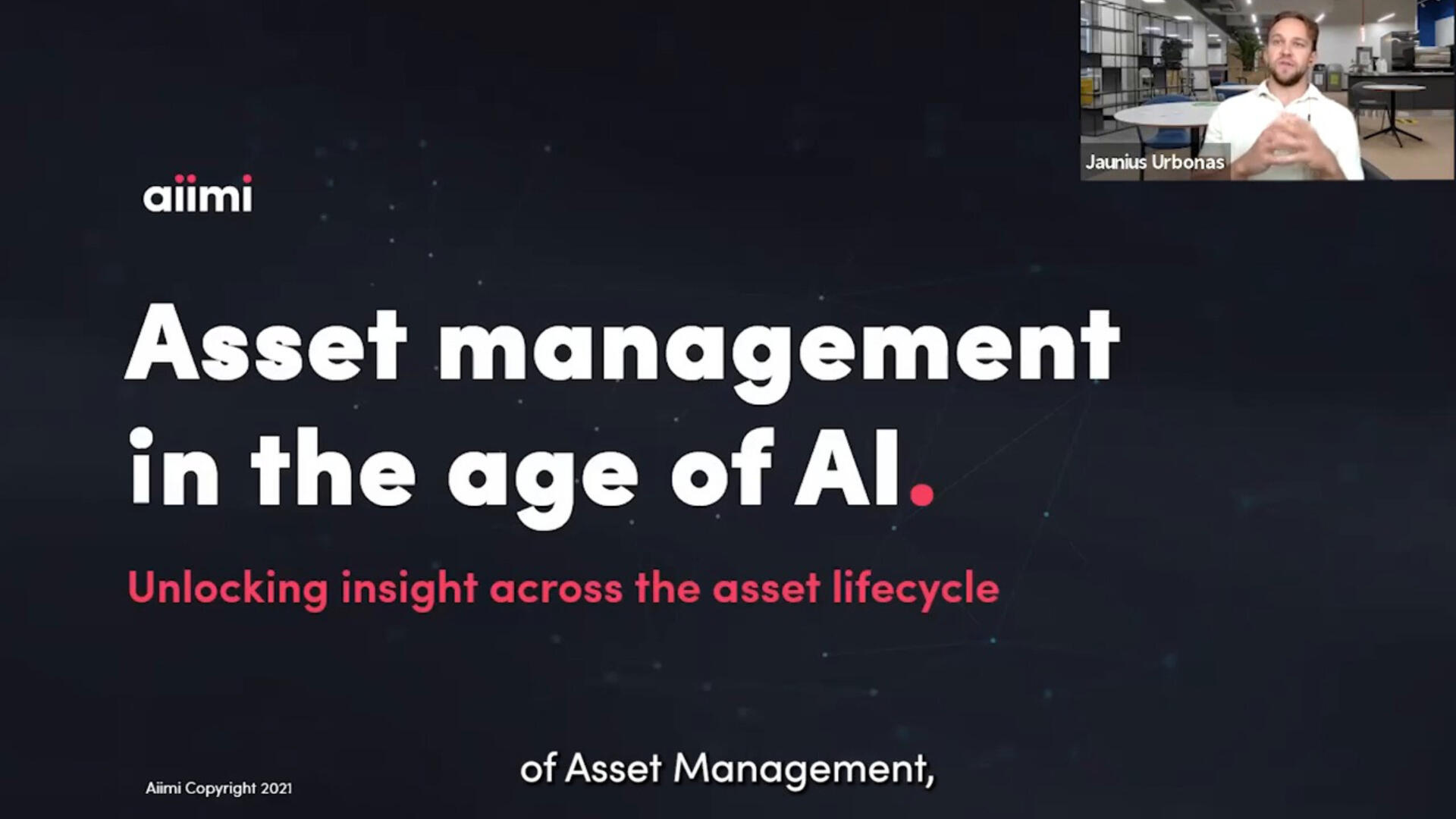
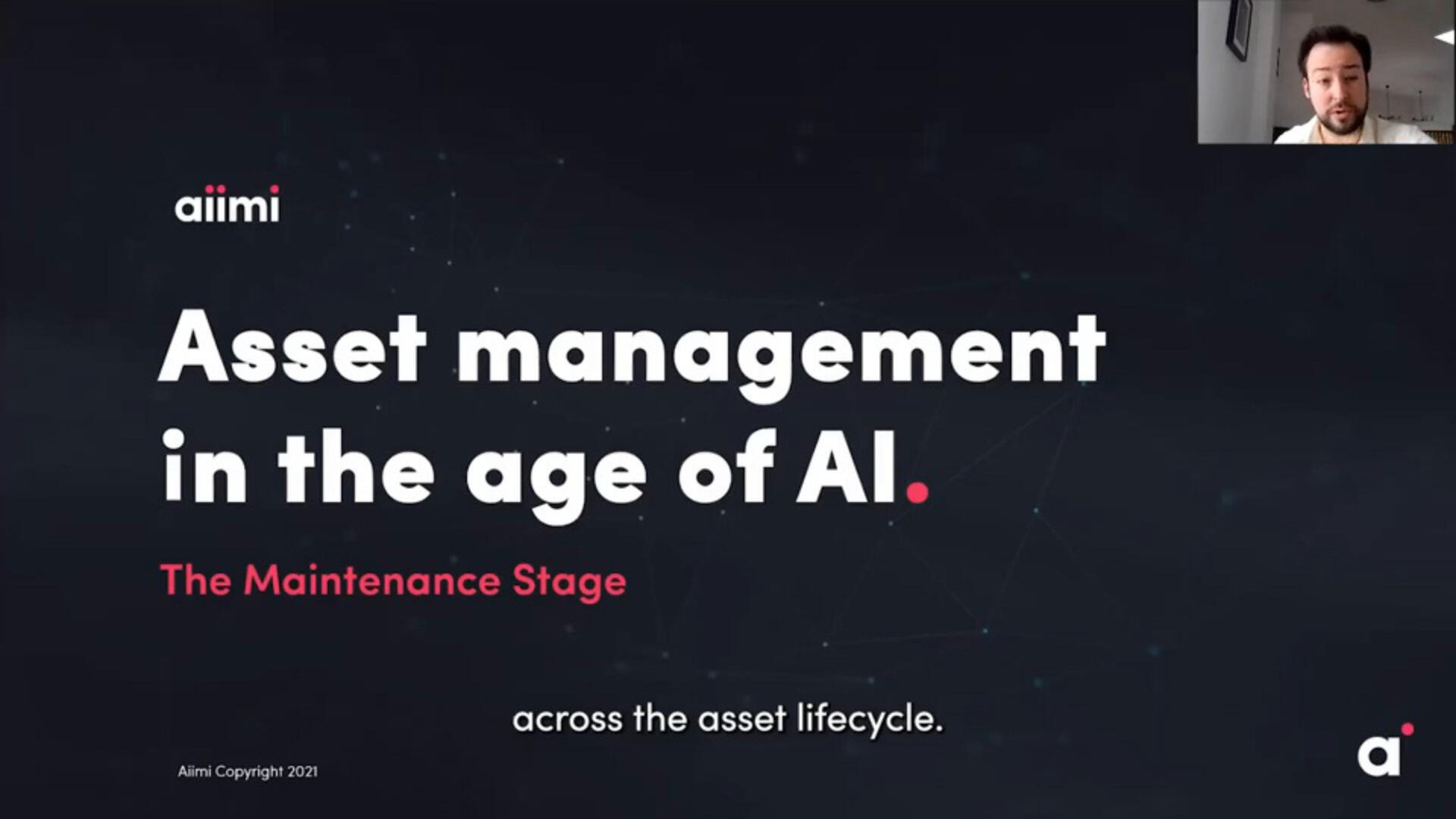
During the design, build, operation, and maintenance of your asset, valuable information is constantly being generated. So, when it comes to asset management, it follows that all data surrounding your asset is as invaluable as the physical asset itself. But when it’s siloed and disconnected, and disparate teams are working on distinct stages of the asset lifecycle, how do you continuously access, discover, interconnect, and optimise high quality, low cost, data-driven business value?
Data and information are equal partners – stronger together
When organisations collect and connect their data and information, they need to treat them equally, recognising that collective, interconnected content delivers far more value. The answers to all your questions are somewhere – hidden in documents, emails, spreadsheets, models, data analytics, transactions, telemetry data, CAD files, PDFs, and CSV files – but they’re not always stored in one singular place.
By joining the dots, extracting, or deriving new data points, and surfacing new relationships, the overall value of that data grows. You can more easily find things, reducing rework and duplications, while ensuring that nothing gets missed. And when you’ve landed on something useful, you can see everything else that’s connected to it, adding even more context. This enrichment allows you to consume and deliver insight to help inform your future asset designs, builds, operations, and maintenance, which previously would not have been possible.
Automate information governance – stay out of compliance hot water
Organisations often implement tools to help govern their information and stay compliant. Mostly, these are ineffective because the speed and volume of data generated and the increasingly complex structures, forms, size, and velocity of data are too hard to easily manage. Plus, as soon as the data science model and enterprise architecture are complete, the tech changes.
With regulatory compliance being key to delivering your asset project – and mindful that data sits at the heart of it – you need to automate your information governance wherever possible. Here, natural language processing (NLP) and machine learning (ML) step up to the challenge, automatically discovering information and extracting metadata to automate governance, while AI polices compliance and tracks everyone in following the rules.
It’s all about the user experience – connections in context
The user experience needs to take centre stage. People with distinct roles across asset management need to work and collaborate in ways that make sense to them, so you need to understand your end-to-end user journeys – and tech can pave the way. By automatically crawling, discovering, classifying, and interconnecting information across your enterprise systems, AI solutions such as insight engines will simplify your search and discovery. Automatically geotagging and visualising your information on data maps and dashboards will also help your users to address your asset-centric challenges.
Design and build – digital twins and insight engines
The design stage offers the perfect opportunity to brainstorm ideas and take the opportunity to tackle your biggest asset-centric challenges. Collaborating with cross-functional teams for a user-centric approach will help you tap into the knowledge and experience of a broad section of professions across your entire enterprise. By teaming up for agile ideation exercises and assessing your solutions with real users, you can develop user-validated prototypes, strategise how to redesign your underperforming assets, and weigh up the cost-benefit ratio.
By treating data and information as two sides of the same coin at this stage, you will unlock a more holistic digital view of your assets and build solid foundations for a digital twin. As a virtual real-time representation of your physical asset, a digital twin will only be as good as the combined content it’s built on. Pipeline data capture and telemetry are explicitly planned to capture sufficient information to build an exact, near-real-time digital twin. And because two projects – real and digital – are running concurrently, both systems more accurately capture information on asset specifications than when relying on legacy data. It’s far easier to get the systems and processes needed for a digital twin right first time, as part of your design and build phases, than it is to retrofit a digital twin into existing infrastructure. Although AI, of course, can still benefit your existing assets.
And rather than storing your data, legacy and new, in a centralised system to share and govern it, you can use an insight engine to find document types and hidden data, such as site codes and asset types, with ease and without moving data out of source systems. This way, your front-end users can search existing asset data for technical details across several systems to find what they need, exactly when they need it, accelerating your asset design and build.
Operations – data scientists and visual dashboards
Your asset teams need to carry out complex data science projects at the operations stage. It’s not humanly possible to manually check the constant stream of data supplied by your sensors and equipment. That’s where an AI platform, like an insight engine, can automatically scan for patterns and problems constantly, accurately, and quickly.
Inviting a data scientist to the table early on will also help you consider your challenges from a unique data-driven perspective, while agile sprints with users, consumers, and stakeholders will prevent costly iterations, fine tuning data pipelines and visual dashboards to resolve issues, make predictions, and view anomalies alongside relevant data.
Maintenance – harnessing AI for effective scheduling
One obvious win stemming from high-quality operational data science models is reduced false-positive reactive maintenance. However, greater understanding of asset health in the first instance can help inform preventative maintenance, resulting in fewer unscheduled measures and costly asset failures. But exactly how do you stay in control of scheduled maintenance?
First, you need to optimise your schedule, which requires careful team collaboration to weigh up each scheduled maintenance task and data analytics to ensure optimal team utilisation. Second, use algorithms to form a data science technique called clustering, dividing data points into several groups. Third, highlight these clusters with visual data maps to help your asset team understand the scope of work suggested by your data science model. Visualisation drives business adoption.
As patrons of the Institute of Asset Management, we'll be at the IAM UK Conference 2021 at the Chelsea Harbour Hotel, Stand 10. Come and chat to our Data Science Principal, Jack Lawton, and Account Director, Steve O'Donoghue, about all things data and AI in asset management.
Aiimi Insights, delivered to you.
Discover the latest data and AI insights, opinions, and news from our experts. Subscribe now to get Aiimi Insights delivered direct to your inbox each month.
Aiimi may contact you with other communications if we believe that it is legitimate to do so. You may unsubscribe from these communications at any time. For information about our commitment to protecting your information, please review our Privacy Policy.
Enjoyed this insight? Share the post with your network.
Content Writer
Vienna delivers an effortless and insightful user experience

Eversholt Rail adopts Aiimi Insight Engine to deliver advanced business insights

How to apply AI for Business Intelligence (an ideal first GenAI use case)

The benefits of data governance in the age of AI: delivering trust, supporting innovation

The rise (and risks) of Edge AI
