Good data is one of your greatest assets - How to measure and improve data quality to drive your business forward.
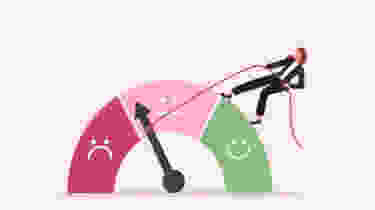
A harbinger of digital transformation on a global scale, data has crept into the length and breadth of our organisations. Driving our business decisions and shaping our growth strategies, actionable data insight hangs on high quality data. Aiimi Data Engineer James Robinson gives business leaders the low-down on why measuring data quality matters – and how to bring technology to the table to drive improved data quality.
Eagerly stockpiling high volumes of data, your transactional systems and analytical databases continuously capture your day-to-day data and store your key datasets. A mountain of information, they steer your business intelligence above simple reporting and beyond predictive analysis.
Today, data maturity is so advanced that integrating it into your data reporting or machine learning (ML) models unlocks fresh new data insight, driving key business decisions. But although ML is ravenous for data, it’s a discerning diner. Afterall, ‘you are what you eat’, or as the data science community puts it, Garbage In, Garbage Out.
What’s the gig with GIGO? And why does data quality matter?
Garbage In, Garbage Out (GIGO) is intrinsic to all data science projects. Even if the world’s most preeminent data scientists created a first-rate machine learning model for your business, feeding it high quantities of low-quality data would render the results worthless.
In essence, GIGO emphases the importance of high value data when applying ML models to business use cases. And this is elemental when you’re making business decisions off the back of it. Collecting vast amounts of mediocre data won’t generate the results your business needs, so it’s important to be able to measure data quality effectively to direct your clean-up efforts and identify foundational errors, well before you start pumping data into your ML models.
Five key metrics to help you measure data quality
Ensuring your projects are powered by good data is crucial, but before you embark on your journey to seek out good data for your priority use cases, let’s clarify a couple of things. What exactly does good data look like? Quality over quantity is key to doing data better. But what is high quality data, and why it is so important for generating genuine business value from your machine learning and data science projects? Essentially, good data boasts five key quality metrics:
- Accurate – you need to avoid any errors in your transactional data (e.g., sales orders, invoices, shipping documents, credit card payments, and insurance claims), and establish a contingency plan to fix any inaccuracies that are inputted into your transactional systems.
- Relevant – data quality must be relative to the purpose you’re using the data for. Onboarding a subject matter expert to work alongside your data team will focus relevant data collection to meet your needs. And to meet your needs, you need to define its intended use. This also ticks a key GDPR requirement.
- Coherent – your data should be comprehensible to your data team, and every final dataset should make sense to your end users to avoid misuse and miscalculations further down the line.
- Complete – missing data can introduce bias into your decision making because it creates an incomplete picture. You need to address potential omissions – gaps in the datasets you’ve collected – and check that you’ve collected every single related dataset.
- Accessible – your data needs to be secure yet easily accessible to those who need it
– that means cataloguing and making it clear to business users what data is available to them
Developing the foundations for high quality CRM data at Kinleigh Folkard & Hayward (KFH)
When building new systems, platforms, or processes, it’s important to keep one eye on how business users will create and maintain high quality data. By shedding light on how your data teams collect, store, analyse, and report on your data and information, you’ll develop a good understanding of your current situation and help identify any barriers that are likely to hinder data quality.
For example, in collaboration with Kinleigh Folkard & Hayward (KFH), Aiimi helped the estate and property service provider completely overhaul its residential sales CRM (Customer Records Management) system with a powerful custom-built data platform.
When building new systems, platforms, or processes, it’s important to keep one eye on how business users will create and maintain high quality data.
But before we could start thinking about developing their new user-friendly platform, it was vital that we first focused on what constitutes a good customer data record for the business, so that we could make sure their new system was perfectly designed to capture the best quality data.
Next, we analysed KFH’s current data against our five key quality metrics: is your data accurate, relevant, coherent, complete, and accessible? This revealed a data quality score, which not only helped the data team who were designing the data cleansing pipelines for the historical data, but also kept the digital team in the loop. This meant the digital team knew exactly what rules to apply to their new front-end OpenHouse NextGen system.
Developing this Data Foundation means that KFH can now rely on integrated, trusted, and timely data to use within reporting and analytics across business use cases – and the key metrics for measuring data quality are now embedded in the business and its systems.
Remove manual error and improve data quality with automation
Data analysis is an important part of any organisation’s business decision making, but some of the most common data formats used for these processes often come with a multitude of problems. For example, spreadsheets are often populated with data taken from isolated extracts – derived from databases – before they’re intricately analysed. Although common practice, this makes it difficult for businesses to pinpoint exactly what data’s being used and when, how it’s being transformed, and who has access to it. Plus, it can be tricky to spot mistakes and differences between cell formulae in spreadsheets, making it easy for errors and mistakes to sneak in.
As there’s no easy way to find and manage what’s been created and how it’s been generated using manual systems, standardising, unifying, and sharing data across your business become more difficult. Several different teams could unknowingly be carrying out work to address the exact same issues, not only duplicating effort but potentially using slightly different definitions of data and data transformation logic. Ultimately, manual processes and reduced visibility make it hard to determine one single version of the truth (SVOT), which is a massive barrier to maintaining high quality data standards.
Another place where data quality can slip is human interaction. This can happen at any point in the manual data inputting process. Often menial and repetitive, it’s easy for focus to drop and mistakes to crop up when data must be manually processed or entered by your users. Any manual interaction with your data is an area that should be marked as a possible point of failure, and while validation may mitigate against error, manual interaction should be avoided unless strictly necessary.
Manual processes and reduced visibility make it hard to determine one single version of the truth (SVOT), which is a massive barrier to maintaining high quality data standards.
So, how can you overcome these problems and ensure only high quality data assets make it into the right hands? By focusing on creating automated data pipelines, your data teams can replace the need for formats like spreadsheet exports or manual data input processes. Automation eliminates any chance of human error and creates one SVOT, so all your teams are working from the same page. You’ll also free up your data team’s time to generate new data insights to help move your business forwards.
As well as automated data pipelines, AI-powered technology like Insight Engines can transform how your data teams and business users access information. By automatically indexing and interconnecting all data sources, an Insight Engine can create a fully-searchable data mesh that’s always up-to-date, complete, and accurate. That means you don’t need to bring your datasets together onto a single, centralised data warehouse (Cloud or on-premise) – data can reside locally in a decentralised data landscape, with the data mesh connecting users to insights. Equally, data already housed centrally can form part of the same data mesh, acting no differently to other source ‘nodes’. With data mastered just once, duplication is eliminated and generating insights becomes more efficient and effective.
By automatically indexing and interconnecting all data sources, an Insight Engine can create a fully-searchable data mesh that’s always up-to-date, complete, and accurate.
Use high quality data to address your biggest business challenges
Now you know why good data matters, how we can measure data quality, and why automation and AI technology are key to ensuring your data teams can access the highest quality data with ease. All that remains is to ask, what business challenge have you set your sights on next, and how can you drive it forward with data?
Find out how some of the UK’s largest organisations are harnessing data and AI to transform strategies, generate new insight, and achieve their goals. Read our Case Studies.
Aiimi Insights, delivered to you.
Discover the latest data and AI insights, opinions, and news from our experts. Subscribe now to get Aiimi Insights delivered direct to your inbox each month.
Aiimi may contact you with other communications if we believe that it is legitimate to do so. You may unsubscribe from these communications at any time. For information about our commitment to protecting your information, please review our Privacy Policy.
Enjoyed this insight? Share the post with your network.
Top Trends in Data & AI for 2021: data mesh

2020 grading algorithm: understanding what went wrong
