The definition of insight.
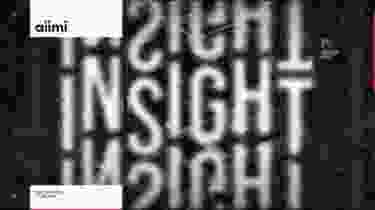
Aiimi’s mission is to connect people to insight, because we believe insight is what separates the good from the great. Used to its full potential, insight can help you avoid failure, find opportunity, mitigate risk, increase efficiency, and gain competitive advantage.
So, what is insight – and where do you find it?
Insight is what you get when you bring multiple datasets together to reveal something greater than the sum of its parts. It’s interesting, valuable, and only made visible when you join up the dots between data.
But insight is only as good as the data itself, and to get good quality data, you need good data governance. A data governance strategy creates the rule book where a company agrees on how to describe, own, and manage data, from when it’s created to when it’s deleted. Once you’ve discovered, classified, and labelled data to describe it in consistent terms, you can start to interconnect it and get insight.
The interconnected view
In the consumer world, insight can help companies build a clearer picture of their customers. I was once involved in a project where Tesco and Visa were both seeking to better understand their customers and their spending habits. Tesco (via their data company Dunnhumby) drew most of their customer data from their Clubcard scheme, which provides an itemised breakdown of each customer’s purchases. When I use my Clubcard, Tesco knows I’ve bought pizza, nappies, and a case of beer. Meanwhile, Visa knows how much I’ve spent at Tesco, but doesn’t know which items I bought. What Visa does know is that five minutes later, I drove past Tesco’s petrol station and filled up at the Shell garage down the road. They also know I went to the local florist and a butcher on my way home.
By collaborating and bringing together those data sets, the two companies were able to get valuable insight into their shared customers and use it to take action. With a more complete picture of me and my spending, Tesco could start targeting me with offers to gauge my price point for buying flowers, meat, and petrol, and encourage me to spend more in store. Visa can build a richer picture of its customers, and segment them for more relevant incentives that will help retain them and increase wallet share.
If joined-up data enables insight, then data silos present the first barrier to insight. If we look at Local Authorities, for example, there are hundreds of departments across benefits, parking, libraries, access to healthcare, adult and children’s services, and so on. Each of these departments will use its own separate system, mostly because that’s just how things have evolved, and perhaps partly because of security concerns. This means the data in each system is usually only accessible to one department, so each team only gets a partial view of their customer, and the customer gets a disjointed experience.
But if we were to connect up these disparate datasets within a citizen label, we’d get a complete picture of each person and could start to serve them better. We could then automate processes throughout real events that happen in people’s lives: when somebody moves into the area, we could automatically invoke the relevant services, such as setting up council tax, applying for residential parking permits, and registering them with a GP; when someone approaches school age, we could trigger services around education; if a vulnerable person is identified, we could check if they’re eligible for benefits, trigger the necessary support from social services, and signpost them to relevant funded third sector services. Joined-up data gives us a more complete citizen profile, so we can automatically trigger actions and provide a far better experience.
Applying insights to mitigate risk and failure
The potential for insight lies in pots of data within separate systems, and it only becomes discoverable and visible when we start to interconnect the data. When things go wrong in an organisation – a job isn’t fulfilled, health and safety is breached, or the customer experience is poor – it’s most often because systems or departments aren’t connected to each other. The flip side of this is that when Aiimi goes into an organisation looking to drive productivity or efficiency, this is the first place we look, and where we can have the biggest impact.
If interconnected data can have a positive impact on every task an organisation carries out, it becomes a game-changer at enterprise scale. Anglian Water undertakes millions of scheduled maintenance jobs a year. When a job needs doing, it isn’t enough to pull any available worker from a roster – with joined-up data, Anglian Water can ensure it matches the right person to the right job, with the right skills and certifications, in the right location, with the right tools in their van, and the right Health & Safety briefing, so that every job can be fulfilled completely and compliantly first time.
Fraud detection is another great example of how insight can be used to mitigate failure. I worked with a major retailer years ago who were having issues around returns. In a region of 100 stores, two or three were noticing an unusually high number of returns for high-value products. Looking at the data, they were effectively outliers compared to the returns in other stores – and wherever there’s an anomaly, that’s the place to take a closer look. By connecting the returns data to point-of-sale data and HR rotas, we were able to see who was on shift when the returns took place. This gave us clear sight of the individual responsible, enabling an investigation into whether this was a training issue or in fact fraud.
Scaling up insight with automation and analytics
When we automate pattern-spotting to get insights, we’re able to do it on a much bigger scale, with many more data sets, meaning we can find more interesting insights that wouldn’t otherwise be available. For example, to help regulate financial organisations by revealing connections between Companies House, company directors, and payments made to other individuals and organisations. When we visualise these results, we get a heat map that identifies anomalies in cash flow or payments that deviate from typical patterns, and can trigger an alert for investigation.
In asset-heavy industries like engineering and utilities, the impact of joined-up data and insight is clear. There are veteran engineers who can walk around a water treatment plant, place a hand on a piece of equipment, and by sensing its temperature, vibrations, noise, and smell, automatically tell you if it’s running OK. But this isn’t scalable; there are very few people who can do it, the information isn’t easily shared, it requires physical proximity to the asset, and it’s not necessarily accurate. By bringing together all the data points we have, we’re able to make the insight accessible to everyone who needs it. And by monitoring patterns in performance, we can also start to make predictions, spot indicators of failure, and invoke reactive pathways to de-risk. You couldn’t get any of this from just a single data point.
By connecting data together, we’re able to take a more reactive approach to servicing assets, so we can do the right maintenance job at the right time. This moves us away from planned maintenance cycles, where set schedules mean some assets will be serviced before they need to be, and others will be serviced too late. Insight enables us to work smarter, giving us an informed view of what maintenance will be needed and when.
Insight and AI, underpinned by data quality
A lot of insight comes from good old-fashioned analytics – this is still a massive opportunity, and one that’s often underplayed amid the hype around AI. We’re enterprise AI advocates at Aiimi, but we also know that AI is only as good as the data it’s given. The more high-quality, interconnected data we give to AI, the better it’ll perform for us. So, while AI isn’t the final answer in and of itself, it is another driver for data quality.
Data quality is crucial in even the simplest data capture, such as customer names. A misplaced character is the difference between Steven Salvin and Stephan Salvin, or you could trip over an abbreviated version such as Steve Salvin or S. Salvin. Already, my presence as a customer has been diluted by a factor of four. We often see this in financial services, where a slight difference in credit card details versus mortgage details can make for repayment irregularities. A customer will believe they’re known across an organisation, and it’s easy to assume these organisations have this level of capture nailed, but it's a real problem.
I first encountered this when working in insurance; claimants would deliberately tweak minor details of their applications in order to make multiple claims, and it wasn’t obvious that these claims were all from the same person. This was most common in whiplash cases – applicants would make multiple claims by applying as the secondary and tertiary passenger in other accidents. We had to start looking at other data points to prove that they were all in fact the same person, by triangulating things like phone numbers and addresses. In this case, connecting data helped to detect fraud, but it can also lead to better customer service.
When you leave data in silos, you’re exposed to the risks of things going wrong – like fraud, non-compliance, and poor customer service. But when you join it up, you don’t only avoid those failures – you also open up additional opportunities for efficiency, cost-cutting, improved performance and customer experience, and better AI outputs.
Gaining insight is about linking data together. It’s the way we make data work more universally and join the gaps between business functions – it’s the value we can only start unlocking when we interconnect all enterprise data to give us the entire data picture. And it all starts with good data governance. High-quality data means greater insights and better business outcomes, including valuable AI outputs. In other words, when we get data governance right, we get more insight and value from everything else.
Aiimi Insights, delivered to you.
Discover the latest data and AI insights, opinions, and news from our experts. Subscribe now to get Aiimi Insights delivered direct to your inbox each month.
Aiimi may contact you with other communications if we believe that it is legitimate to do so. You may unsubscribe from these communications at any time. For information about our commitment to protecting your information, please review our Privacy Policy.
Enjoyed this insight? Share the post with your network.
The rise (and risks) of Edge AI

How CIOs can shape business strategy—by choosing the right tech
