Enrichment: Giving users what they need.
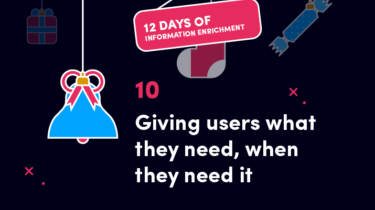
Over this series, I have talked a great deal about how we perform enrichment steps. In this blog, I want to look at how we use this enrichment - along with other data that InsightMaker (our AI-powered discovery and insights platform) collects - to provide users with recommendations.
Recommendations in retail, and beyond
In your everyday life, you probably see recommendations being used all the time and by all sorts of web platforms - from media and streaming services like Netflix, to shopping sites like the giant Amazon. This may seem like a world away from the types of things that we want to do in a business context, but, if we take the time to look at the underlying algorithms, it becomes apparent that we can use these same approaches. In fact, if we take our extended knowledge of our employees and overlay this on top of some of these established recommendation algorithms, we can end up with some pretty cool and indeed accurate approaches to providing business users with what they need when they need it.
Let's quickly look at some basic approaches to providing a recommendation. To do this, I want to use an example from the retail world. In retail, we want to try to determine which products an individual may be interested in and then promote these to them, perhaps via email or an in-place advert online.
One basic approach to this problem is to examine what an individual has previously purchased, look for sets of people that have purchased the same items, and then recommend the items that they have purchased which our individual has not. This is based on the simple assumption that, if individuals share similar purchasing patterns, they are likely to be interested in the same additional items.
Of course, when analysing clusters of similar products, we want to filter these by type - for example, home entertainment. After all, just because Bob and Peter purchased the same TV, does not mean that Bob is interested in the beard trimming kit that Peter purchased last week.
Using recommendations to help your employees
So, how can we apply this in a business context? How can we use these techniques to provide valuable and meaningful recommendations to business users? Well, the one great thing that we have plenty of is knowledge about our users. Their job role, their responsibilities, and - if we have access to things like their calendar - even what they are doing at specific points in the day. Better still, we also have data in InsightMaker that tells us what users have been looking for and what documents they have looked at.
Take the example of a field engineer tasked with fixing an asset. To serve this engineer useful content recommendations, we may look at the content that previous engineers have used along with the content that our engineer has looked at, and then try and determine some key items that should be relevant. For instance, it may be that there's a crucial piece of health and safety information which everybody except Barry, our field engineer, has looked at. Clearly, it would be very useful for Barry if we could recommend this document to him. What we have done here is to apply similar techniques to our retail example, but to overlay them with what we already know about the user and what they are about to do. This means we can give Barry contextualised recommendations. It’s this contextualisation that is so crucial to making relevant and useful recommendations.
Another example is clustering content to find authors who produce similar content, or by extension, people that are experts or engaged in similar activities in the business - for example, designing specific parts or systems in a car. This enables us to provide people recommendations, rather than content recommendations. Being able to connect like minded people together in the business can be a very powerful concept. Taking this a step further, you could also recommend to these users more documents that other expert users other have written which contain similar concepts and topics.
Making recommendations work in the business presents some very exciting opportunities. It’s something that we are actively working on at the moment in Aiimi Labs, and we look forward to completing our first feature set in this space early next year.
Cheers and see you soon, Paul
If you missed my blogs in the 12 Days of Information Enrichment series, you can catch up here.
- Day 1 - What is enrichment? Creating wealth from information
- Day 2 - Starting at the beginning with Text Extraction
- Day 3 - Structuring the unstructured with Business Entity Extraction
- Day 4 - Solving the GDPR, PII and PCI problem
- Day 5 - Sustainable Document Classification
- Day 6 - Image Enrichment: Giving your business vision
- Day 7 - Advanced Entity Extraction with Natural Language Processing
- Day 8 - Understanding customers with Speech to Text translation
- Day 9 - Accelerating classification with Document Clustering
- Day 10 - Giving users what they need, when they need it
- Day 11 - Understanding documents with Dynamic Topics
- Day 12 - The power of Enrichment
Aiimi Insights, delivered to you.
Discover the latest data and AI insights, opinions, and news from our experts. Subscribe now to get Aiimi Insights delivered direct to your inbox each month.
Aiimi may contact you with other communications if we believe that it is legitimate to do so. You may unsubscribe from these communications at any time. For information about our commitment to protecting your information, please review our Privacy Policy.
Enjoyed this insight? Share the post with your network.
How to apply AI for Business Intelligence (an ideal first GenAI use case)

Llama 2: Our thoughts on the ground-breaking new large language model
