Enrichment: Structuring the unstructured with Business Entity Extraction.
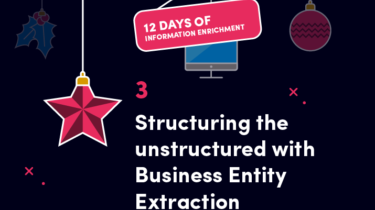
In the previous installment of 12 Days of Information Enrichment, we discussed how we go about extracting text content from the vast array of document formats that are found in the enterprise - and how we do this in an efficient, scalable and reliable way.
Next on the agenda is how we begin to add additional business context to information, fundamentally changing the way in which we can utilise it. From better navigation and discovery, to reporting on what we do and don’t have, to being able to use our information in a data science and machine learning scenario - business entity extraction is a great place to start.
The three main methods of entity extraction
Adding additional context can manifest itself in a variety of ways; the one we are going to focus on here is specifically how we extract key pieces of metadata from within information, and then store this as a series of labels. Quite often, these pieces of metadata will be business entities such as a customer number, asset number or an SAP functional location, but they could also be things like geotags.
Generally speaking, there are 3 ways to extract entities from information - dictionary-based, regular expression and statistical named entity recognition.
Dictionary-based entity extraction works by using a list of known terms that we are interested in, making it ideal for master data references. Regular expression entity extraction uses lookups to capture entities that follow a strict format, for example an asset number or a customer reference number. Finally, statistical named entity recognition utilises trained models that understand language structure, meaning that they are great for things like people's names or locations.
From working with organisations, we quickly realised that dictionary-base and regular expression entity recognition offered the biggest business benefits and covered the vast majority of what we were interested in. Statistical, whilst good, simply required too much training of an organisation's information to build a domain specific model, compared to the level of benefit it would deliver.
Taking our own approach to entity extraction
We looked at various implementations for entity extraction, including some Open Source options. However, many of these were heavily optimised for statistical entity extraction and were slow and expensive for dictionary-based and regular expression entity extraction. So, we took the plunge and decided to craft our own entity extractor that would be super-fast and scalable! We called this the Trie entity extractor and we use it within our information discovery platform, InsightMaker.
Internally, this entity extractor was heavily optimised to construct the dictionary of business entities so that we could extract all entities with a single parse of the text. In addition, we were able to handle regular expressions at the same time and very efficiently extract email addresses from content. Another key design consideration was how it would perform over tens and even hundreds of millions of documents. This crucial feature led us to undertake countless rounds of performance testing and optimisation.
Extending on this capability, we then looked at ways to use our extracted entities to map to things like geotags. Mapping to geotags allowed us to associate documents and data with the actual geographic location of assets or sites, for instance, and then use these to present the information to the user though mapping and GIS technologies.
So, quite a simple enrichment step, yet an incredibly fundamental one when it comes to adding business context to information and being able to transform the way we use it. Furthermore, being able to do this at lightning speed really matters when working at an enterprise scale dealing with hundreds of millions of documents.
Next time, we will be looking at how we bring these entities together to help solve the problem of detecting Personal Identifiable Information and Payment Card Information to comply with legislation such as GDPR.
Cheers, and see you soon, Paul...
If you missed my blogs in the 12 Days of Information Enrichment series, you can catch up here.
- Day 1 - What is enrichment? Creating wealth from information
- Day 2 - Starting at the beginning with Text Extraction
- Day 3 - Structuring the unstructured with Business Entity Extraction
- Day 4 - Solving the GDPR, PII and PCI problem
- Day 5 - Sustainable Document Classification
- Day 6 - Image Enrichment: Giving your business vision
- Day 7 - Advanced Entity Extraction with Natural Language Processing
- Day 8 - Understanding customers with Speech to Text translation
- Day 9 - Accelerating classification with Document Clustering
- Day 10 - Giving users what they need, when they need it
- Day 11 - Understanding documents with Dynamic Topics
- Day 12 - The power of Enrichment
Aiimi Insights, delivered to you.
Discover the latest data and AI insights, opinions, and news from our experts. Subscribe now to get Aiimi Insights delivered direct to your inbox each month.
Aiimi may contact you with other communications if we believe that it is legitimate to do so. You may unsubscribe from these communications at any time. For information about our commitment to protecting your information, please review our Privacy Policy.
Enjoyed this insight? Share the post with your network.
How to apply AI for Business Intelligence (an ideal first GenAI use case)

Llama 2: Our thoughts on the ground-breaking new large language model
