Enrichment: Sustainable Document Classification.
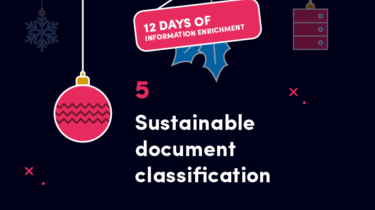
Document classification. Two words that will make you think of records management, classification trees, retention schedules and relentless user adoption programs where you resort to begging people to correctly classify their content because, if they don’t, the whole thing was a great big waste of time and money.
But it’s not just records management that’s a driver for classification nowadays. Document classification can be a crucial enabler for your cloud strategy, telling you what things you can and can’t put in the cloud. It can drive more useful search and navigation for users. And it can also underpin your information security policies.
For Day 5 of 12 Days of Information Enrichment, I want to tell you about some interesting work that Aiimi Labs are doing to automate document classification. We want to make document classification more sustainable for organisations as part of our enrichment capability.
Document classification - the old way!
Many approaches to document classification require users to manually select a bunch of things when adding a document. In fact, if you go and have a look at some old school document management systems it’s no surprise users get fed up with it, I mean there are literally more fields to fill in than a tax return. Yes, I am exaggerating, but you catch my drift!
Some advances have been made using machine learning to build document classification models. However, they require extensive training, and this requires people to manually label enormous volumes of training data - a huge blocker.
So, how about if we could automate the training of document classification models? That would be pretty cool, right?
Document classification using machine learning
At Aiimi Labs, we have been working on a series of techniques that allow us to use machine learning, specifically unsupervised clustering, to examine large collections of documents and tell us the groups or classifications that exist within them. It’s called ‘unsupervised’ because it does not need people to train it – you just let the machines get on with it and tell you the answer.
Once you have the clusters of documents you can then examine these, work out what the clusters represent (for example, invoices or purchase orders), and build your classification model from that.
Of course, you may have some errors and a handful of things may get misclassified along the way. To help eliminate this, we use confidence scores which assign a numerical score indicating how confident the system is in its classification, as well as crowd sourcing to help correct misclassified documents.
What’s also great is that we can reinforce the models by continually retaining them on a growing document set, so they get better and better.
So, there you are – a more cost effective and crucially a more sustainable approach to that age-old challenge of document classification, and of course, another very fundamental step in the enrichment process.
Cheers, and see you soon, Paul...
If you missed my blogs in the 12 Days of Information Enrichment series, you can catch up here.
- Day 1 - What is enrichment? Creating wealth from information
- Day 2 - Starting at the beginning with Text Extraction
- Day 3 - Structuring the unstructured with Business Entity Extraction
- Day 4 - Solving the GDPR, PII and PCI problem
- Day 5 - Sustainable Document Classification
- Day 6 - Image Enrichment: Giving your business vision
- Day 7 - Advanced Entity Extraction with Natural Language Processing
- Day 8 - Understanding customers with Speech to Text translation
- Day 9 - Accelerating classification with Document Clustering
- Day 10 - Giving users what they need, when they need it
- Day 11 - Understanding documents with Dynamic Topics
- Day 12 - The power of Enrichment
Aiimi Insights, delivered to you.
Discover the latest data and AI insights, opinions, and news from our experts. Subscribe now to get Aiimi Insights delivered direct to your inbox each month.
Aiimi may contact you with other communications if we believe that it is legitimate to do so. You may unsubscribe from these communications at any time. For information about our commitment to protecting your information, please review our Privacy Policy.
Enjoyed this insight? Share the post with your network.
How to apply AI for Business Intelligence (an ideal first GenAI use case)

Llama 2: Our thoughts on the ground-breaking new large language model
