Where to use Extractive AI vs. Generative AI for enterprise.
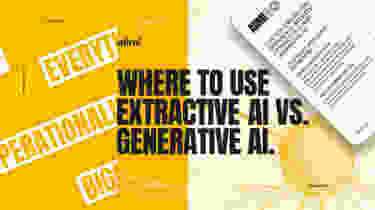
With leaders constantly looking for new ways to increase efficiency and improve employee and customer experience, generative AI for enterprise is having a big moment, thanks in no small part to ChatGPT. But while ChatGPT and now Llama 2 are hogging the limelight (read more about ChatGPT, Llama 2, and how generative AI for enterprise works) we shouldn’t overlook extractive AI. It might be less glamorous, but extractive AI can still be an extremely valuable tool for businesses.
So, when should you use generative AI and when might extractive AI be a better option? Both can be used in tandem with natural language search using a Q&A format, so it’s really all about selecting the best fit for your business use case. In this blog, we’ll share the main pros and cons of each AI technique, so you can bring a balanced view to business leaders and colleagues.
The differences between Extractive AI and Generative AI for enterprise
Let’s start with some definitions…
What is Extractive AI?
Building on the capabilities of NLP, extractive AI can take a user prompt, such as a question, and return a relevant fact or answer from a data set, such as a set of invoices or case studies. This can be very useful to get fast, precise, accurate answers and view them in context within a document. For businesses, this is often exactly what’s needed. However, unlike generative applications, extractive AI can only present back exact text from the source – it can’t create a new response, and if asked the same question multiple times, it should return the exact same answer. If the relevant answer is not contained within the data set provided, extractive AI won’t be able to provide a correct response.
What is Generative AI?
Generative AI can also respond to a user prompt or question by interpreting it and returning an answer in seconds. However, unlike extractive AI, generative AI technology can extrapolate from existing data, reformulating it and even inferring new information. Generative AI is also trained to present its answers back to the user in human-sounding free text (or image, video, or audio form, depending on the tool). This is particularly useful for chatbot applications – like ChatGPT or Llama2 – where the user, such as a customer, relies upon the answer being presented back to them in a very clear format. For more complex prompts, generative AI can be used to summarise coherently, rather than just piecing together extracted segments. This skill, coupled with its ability to generate varied human-like responses, makes generative AI a great choice for many customer service and chat use cases.
Applying Generative AI for enterprise – Open Book vs. Closed Book
Large Language Models (LLMs) are not foolproof when it comes to eliminating bad data from their responses. Though good training and further fine-tuning can hugely reduce risks and ensure that models maintain focus on the intended use case area, accuracy is a challenge when using any sort of generative AI for enterprise.
Leaders can improve the accuracy of generative AI outputs by understanding how to apply generative models correctly – and identifying where extractive AI may be a better option. There are many ways to use generative AI models and these can be applied either in a closed book or open book scenario. Both have pros and cons…
Open Book Generative AI for enterprise
With an open book application of generative AI, the user first has to identify the data or documents for the model to examine each time, e.g. by running a search using their chosen enterprise platform. This drastically reduces the risk of inaccurate outputs, especially if you use a platform like Aiimi Insight Engine, which ensures that your enterprise data is high-quality and well-managed from the outset – and that delivers hyper-relevant search results. This gives a much clearer view of where the model is generating its response from, so user validation is much simpler. This is known as a Retrieval Augmented LLM.
Imagine kicking off a search for ‘engineering team HR reviews’ using your enterprise search platform or Insight Engine; you’ll be presented with a set of relevant documents according to your own access permissions, which you can then pass to a generative model. Using this data alongside a question or prompt, such as ‘summarise the key themes within these employee reviews in under 500 words’, the LLM is neatly constrained to deliver a targeted response.
Closed Book Generative AI for enterprise
In a closed book application of generative AI, the user simply offers a prompt and the answer is derived from the model’s training data set (sometimes called the model’s ‘memory’). Whilst this eliminates a step for users and reduces hurdles compared to an open book scenario, it does limit potential use cases within businesses. In a closed book scenario, there’s no way of setting permissions or controlling access once data and documents have been added into the model, so you must be happy for any data you put in to be visible to users in the form of generated answers. In a large enterprise, where data access is never completely standardised and individual users will have different permissions, this limits use cases to areas like customer service or bid writing, which don’t require confidential or sensitive data.
Plus, with closed book generative AI it’s not possible to get citation for the results you generate, as the model cannot determine which items from its training data set were used to generate a specific result, or where a particular fact appeared within its training data set.
Closed book generative AI applications also bring challenges with retraining. To keep the model up to date, you’d need to be feeding it with new training data at regular intervals, which comes with a cost. Imagine using a closed book application for a bid writing use case – you'd want to be able to draw from your latest and best material to generate new responses.
With both open book and closed book approaches, using a privately hosted model with no connection to the internet (like using the new Llama 2 for business) means your enterprise data doesn’t need to be sent outside the business to be processed by third parties. This is a very important consideration for IT leaders when any kind of sensitive, personal data or commercially valuable data is at play.
Enterprise use cases for generative AI and extractive AI
Generative AI for Customer Service
Customer service is often touted as a key use case of generative AI for enterprises. The simple fact that ChatGPT can generate different answers to the same question replicates the natural responses of humans, making it a useful option for customer service functions. But whilst we want the tool to use different language like humans do, any facts within the responses must still be consistent to avoid misleading or frustrating customers. If not, there’s a danger of creating far bigger customer service issues than those that the model had been intended to resolve.
For some organisations, you may not want varied responses for customer service – consistency can often be far more compelling and useful. In this case, it’s important to consider your model choice, as consistency varies between LLMs. For instance, we’ve found that Llama 2 is far more likely to give consistent answers to the same query, compared to ChatGPT which tends to get creative.
When using generative AI in open book scenarios, tightly controlling the information we give the model to generate its answers from, such as a set of customer FAQs or how-to articles, can also drastically reduce the risks of inaccuracies.
Generative AI for Sales Support
Beyond customer service use cases, there are excellent applications for generative AI in other areas where nuanced or varied free text responses are desired. These could include responding to bids and frameworks, or combining case studies and previous bid responses to generate quality responses within tight word limits. As long as a human can validate the output effectively, there’s great scope for improved efficiency and effectiveness here by automating what can be a time-consuming research and compilation process.
It’s worth noting here, though, that using public LLMs like ChatGPT with enterprise data brings many potential risks, especially in relation to data security and IP protection. In use cases like this, there’s scope for sensitive commercial data to enter the model and leave your enterprise. We’ve discussed the security risks of open source LLMs like ChatGPT for business previously – but our advice is generally to use open commercial models with caution. Instead, look for non-commercial open-source models you can bring in-house and use offline with fine-tuning, to ensure that your data does not leave the enterprise. At the moment, we’re looking to Llama 2 and Falcon AI as potentially viable options for generative AI in enterprises.
Extractive AI for Research and Q&A
Despite generative AI’s impressive capabilities, extractive AI remains extremely useful for enterprise knowledge management. For businesses, extractive AI is a safe option – users can ask a question in natural language, get a response back, and validate that response very easily by viewing it in context. Think of Google’s featured snippets, which provide extracted answers shown in context:
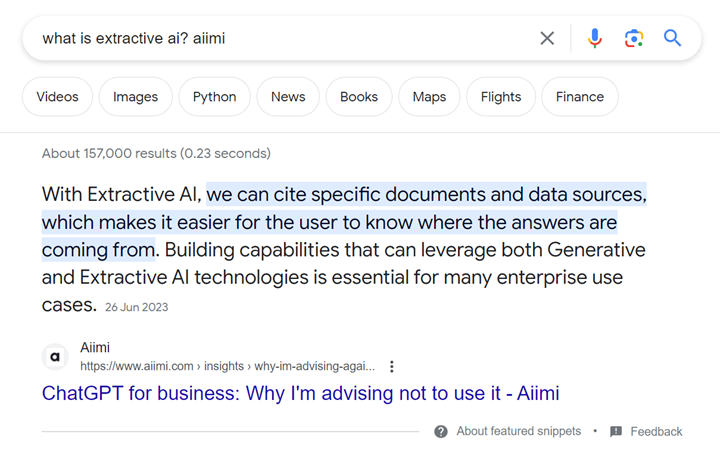
Without lengthy natural language responses, extracted results are often easier to consume and provide a better user experience. The key here is to get the best of both worlds with enterprise AI technology that can call upon both extractive AI and generative AI models, selecting the best tool for the job each time and presenting the results back in an intuitive way.
If you want to extract and summarise information from a specific data set, such as a finance manager querying related customer invoices, or a site asset manager extracting structured data from unstructured PDF site plans, a well-trained extractive AI model is the ideal tool for the job.
Users can still create summaries, created from pieces of extracted content, and natural language can be replicated. But unlike generative AI models, there’s no content generation, so there’s no risk of the model returning data that doesn’t exist. This is particularly relevant when considering legal uses cases, financial data, asset data, and other areas where precision and accuracy is key.
In short, extractive AI is a powerful tool to support natural language Q&A and can be best applied in research use cases.
Generative AI has great potential, but don’t forget about extractive AI
It’s easy to be dazzled by the glamour, potential and sheer ‘cleverness’ of generative AI, but extractive AI still has an important place in your enterprise. It remains an extremely valuable tool for querying data sets and informing summarisation. Where generative AI for enterprise can introduce issues with accuracy, citation, and data privacy, extractive AI techniques offer a safe, transparent option in many cases.
So, look for the big-hitting use cases where generative AI can add real value to your business; to improve process efficiency, to bring consistency and reliability to tasks, or to help you generate better insights, faster. But don’t overlook what extractive AI can do for your business and your users too.
Ready to apply AI in your business? Discover Aiimi Insight Engine, your secure enterprise AI solution.
Aiimi Insights, delivered to you.
Discover the latest data and AI insights, opinions, and news from our experts. Subscribe now to get Aiimi Insights delivered direct to your inbox each month.
Aiimi may contact you with other communications if we believe that it is legitimate to do so. You may unsubscribe from these communications at any time. For information about our commitment to protecting your information, please review our Privacy Policy.
Enjoyed this insight? Share the post with your network.
Content strategist
Four Dimensions of Change: How CIOs Can Integrate Tech, Data, People, and Processes

Gigaclear adopts the Aiimi Insight Engine to enhance data privacy and compliance

How to apply AI for Business Intelligence (an ideal first GenAI use case)

The benefits of data governance in the age of AI: delivering trust, supporting innovation

The Rise (and Risks) of Edge AI
