3 Reasons to Fall in Love With Databricks.
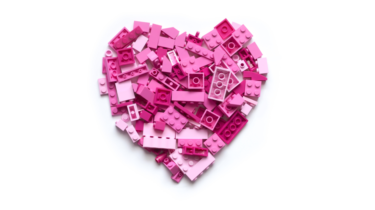
At the moment, I am working to develop an enterprise scale digital twin, from the ground up. When assessing the data required, it very quickly became apparent that our team would have to enter the world of big data (i.e. the volumes were too large for the use of traditional processing techniques). This offered an opportunity for me to finally get my hands on Databricks, an impressive new analytics platform from the creators of Spark which has been rapidly gaining popularity in this area. From the title of this blog, you may have already guessed: I fell in love.
Here are three reasons Databricks stole my heart:
1. Big Data, made easy
I recall vividly, one of my first forays into data science and engineering as a fresh faced graduate was the rather daunting task of setting up a Hadoop cluster. Anyone who has ever tried to set up their own Hadoop cluster will know this pain. Although, in retrospect, it was an excellent learning opportunity, diving into the seemingly endless zoo of Apache projects and configurations was not easy and certainly not quick. Even when following all the guides, tutorials and documentation available, it is almost inevitable that something will not work, and it will not be obvious why!
Of course, the natural solution to these configuration problems would be to use a Hadoop distribution, such as Cloudera. One setup, works out-of-the-box, perfect, right? Well, on one level, yes, but you still have ahead of you the unenviable task of actually writing, testing and deploying your MapReduce or Spark jobs.
Enter, Databricks. Not only does Databricks sit on top of either an Azure or AWS flexible, distributed cloud computing environment, it also masks the complexities of distributed processing from your data scientists and engineers, allowing them to develop straight in Spark’s native R, Scala, Python or SQL interface. Whatever they prefer. Having this development environment directly within Databricks also presents a clear route to production. For even faster development of data science models, Databricks includes MLflow as standard, helping you overcome numerous common hurdles.
2. Notebooks
Ordinarily, I am not a big fan of notebooks. I do not find them intuitive or easy to use as a developer and they are certainly not effective for developing integrated production solutions. However, within Databricks, there are a few details which challenge my long-held beliefs and allow me to accept (and occasionally embrace) the notebook.
- I find the modular cells convenient for exploration. One reality of working with big data is slow calculation speeds and, whilst Spark is the fastest option out there, I often find myself waiting longer than I would like when playing around with my data. Whilst the hidden state shared between cells is commonly regarded as one of the greatest weaknesses of notebooks, I’m glad it is there in Databricks, or I would get much less work done!
- An obvious selling point for notebooks is the in-line graphs and visualisations. In this respect, Databricks is no different. However, what it does have over Jupyter Notebooks on my laptop is that Spark is the only place I can aggregate the large datasets I am working with in the cloud. So, having the ability to drop these visuals in-line in Databricks is a massive bonus.
- Different cells can be in different languages. Whist it may seem like a trivial extension of Databrick’s support for the R, Scala, Python and SQL languages, the ability to have more than one in the same notebook can prove incredibly useful. When working with Spark, I’ve found that solutions which may seem easy at first can prove a little more challenging when they must run as distributed processes! Never before has collaboration been more key, as different developers with different preferred languages may need to work on the same notebook. It’s a welcome change that there are no problems when you need to do one specific thing in SQL, but the rest of the project is in Python.
- Another common criticism of notebooks is that they represent a challenge for versioning and collaboration. This is far from the case in Databricks. It is a pleasure to see that Databricks notebooks enable collaborative working (Google Docs style) and out-of-the-box version control, plus integration with git if you so desire (GitHub or Bitbucket)!
3. Cloud integration
Increasingly, organisations are looking to the cloud. Databricks can be deployed on two of the big cloud providers, Azure and AWS. These integrations turn Databricks from a good tool into a great tool. Immediately, Databricks can take advantage of the scalability, security and production capabilities of these platforms.
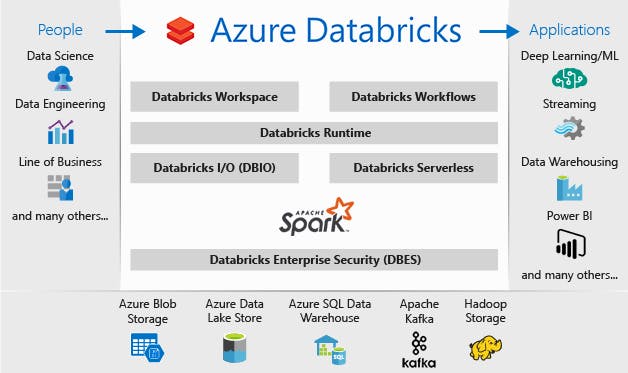
At Aiimi, we’re finding that more of our customers are looking to move their data analytics to the cloud, often using Azure or AWS. You may already be using Azure Data Lake or Redshift for reporting and analytics; Databricks is ideally positioned to hook straight into these existing platforms and push the boundaries of what you can do with your data.
Additionally, using these cloud platforms can supercharge the capabilities of Databricks, for example using SageMaker on AWS. For my current project, I am using Azure Databricks. Two particular Azure services which have helped me to fully utilise Databricks are Data Factory and CosmosDB. Whilst Databricks does have its own scheduling capabilities, integration with Data Factory allows the you to align the triggers and timings of these jobs with various other data operations throughout the Azure stack. Integration with CosmosDB has proven critical, allowing my models to take advantage of a flexible NoSQL database.
My conclusions
Often as a data scientist, I can be reluctant to embrace new technologies which appear to reduce my control and ability to innovate. Databricks keeps the control firmly in my hands. It does not stop me from doing anything – but helps with so much! I can load and explore huge cloud storage datasets with Spark in easy-to-use notebooks, all without having to worry about how I will later adapt this for production. Inbuilt collaboration and version control help keep my code quality high, and I know integrations with Azure or AWS mean that I can plug completed models straight into my existing architecture.
One important point to consider with big data platforms is whether you really need one. There is a temptation to over-engineer solutions for the sake of “doing big data”. However, Databricks not only makes Spark easy, but it fits in so nicely with the Azure and AWS platforms that it can tip this balance – even if you are not quite at big data scale, Databricks is certainly worth a try!
I’d love to chat about your Databricks experiences, or how it could help solve your big data problems. Please feel free to drop me an email with any comments or questions: jlawton@aiimi.com
Aiimi Insights, delivered to you.
Discover the latest data and AI insights, opinions, and news from our experts. Subscribe now to get Aiimi Insights delivered direct to your inbox each month.
Aiimi may contact you with other communications if we believe that it is legitimate to do so. You may unsubscribe from these communications at any time. For information about our commitment to protecting your information, please review our Privacy Policy.
Enjoyed this insight? Share the post with your network.
How data hacks are driving innovation in the water industry

Love lessons: 3 tips and tricks for Databricks users

Benefits of Joining Aiimi’s Partner Programme: A Guide to Success

Aiimi appoints Bytes Software Services as an official referral partner for the Aiimi Insight Engine

Aiimi and Databricks partner to deliver deeper AI and analytics capabilities across shared client base
